In this post, I will walk you through how to build an artificial feedforward neural network trained with backpropagation, step-by-step. We will not use any fancy machine learning libraries, only basic Python libraries like Pandas and Numpy.
Our end goal is to evaluate the performance of an artificial feedforward neural network trained with backpropagation and to compare the performance using no hidden layers, one hidden layer, and two hidden layers. Five different data sets from the UCI Machine Learning Repository are used to compare performance: Breast Cancer, Glass, Iris, Soybean (small), and Vote.
We will use our neural network to do the following:
- Predict if someone has breast cancer
- Identify glass type
- Identify flower species
- Determine soybean disease type
- Classify a representative as either a Democrat or Republican based on their voting patterns
I hypothesize that the neural networks with no hidden layers will outperform the networks with two hidden layers. My hypothesis is based on the notion that the simplest solutions are often the best solutions (i.e. Occam’s Razor).
The classification accuracy of the algorithms on the data sets will be evaluated as follows, using five-fold stratified cross-validation:
- Accuracy = (number of correct predictions)/(total number of predictions)
Title image source: Wikimedia commons
Table of Contents
- What is an Artificial Feedforward Neural Network Trained with Backpropagation?
- Artificial Feedforward Neural Network Trained with Backpropagation Algorithm Design
- Artificial Feedforward Neural Network Trained with Backpropagation Algorithm in Python, Coded From Scratch
- Artificial Feedforward Neural Network Trained with Backpropagation Output
What is an Artificial Feedforward Neural Network Trained with Backpropagation?
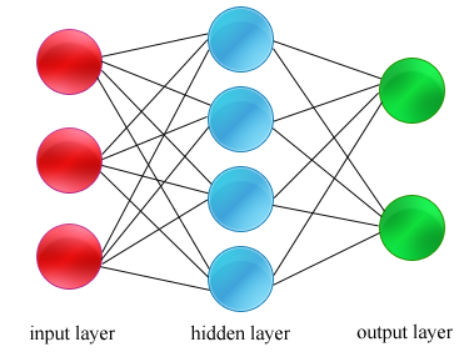
Background
An artificial feed-forward neural network (also known as multilayer perceptron) trained with backpropagation is an old machine learning technique that was developed in order to have machines that can mimic the brain. Neural networks were the focus of a lot of machine learning research during the 1980s and early 1990s but declined in popularity during the late 1990s.
Since 2010, neural networks have experienced a resurgence in popularity due to improvements in computing speed and the availability of massive amounts of data with which to train large-scale neural networks. In the real world, neural networks have been used to recognize speech, caption images, and even help self-driving cars learn how to park autonomously.
The Brain as Inspiration for Artificial Neural Networks
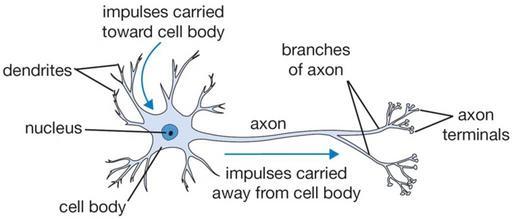
In order to understand neural networks, it helps to first take a look at the basic architecture of the human brain. The brain has 1011 neurons (Alpaydin, 2014). Neurons are cells inside the brain that process information.
Each neuron contains a number of input wires called dendrites. Each neuron also has one output wire called an axon. The axon is used to send messages to other neurons. The axon of a sending neuron is connected to the dendrites of the receiving neuron via a synapse.
So, in short, a neuron receives inputs from dendrites, performs a computation, and sends the output to other neurons via the axon. This process is how information flows through the brain. The messages sent between neurons are in the form of electric pulses.
An artificial neural network, the one used in machine learning, is a simplified model of the actual human neural network explained above. It is typically composed of zero or more layers.
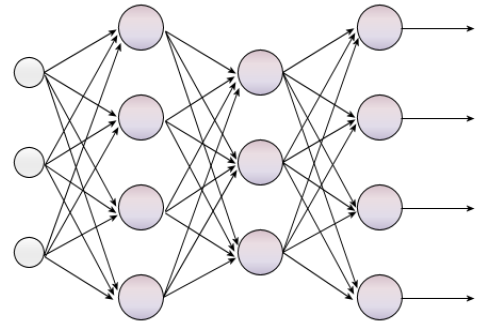
Each layer of the neural network is made up of nodes (analogous to neurons in the brain). Nodes of one layer are connected to nodes in another layer by connection weights, which are typically just floating-point numbers (e.g. 0.23342341). These numbers represent the strength of the connection between two nodes.
The job of a node in a hidden layer is to:
- Receive input values from each node in a preceding layer
- Compute a weighted sum of those input values
- Send that weighted sum through some activation function (e.g. logistic sigmoid function or hyperbolic tangent function)
- Send the result of the computation in #3 to each node in the next layer of the neural network.
Thus, the output from the nodes in a given layer becomes the input for all nodes in the next layer.
The output layer of a network does steps 1-3 above. However, the result of the computation from step #3 is a class prediction instead of an input to another layer (since the output layer is the final layer).
Here is a diagram of the process I explained above:
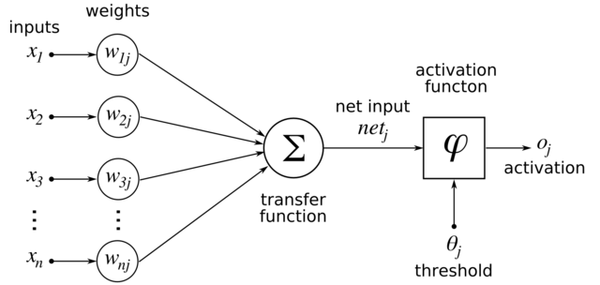
Here is a diagram showing a single layer neural network:
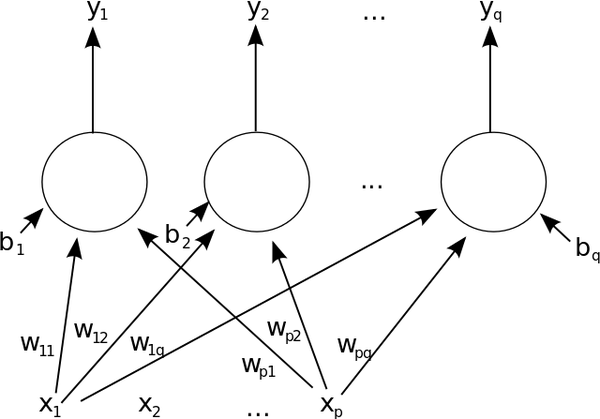
b stands for the bias term. This is a constant. It is like the b in the equation for a line, y = mx + b. It enables the model to have flexibility because, without that bias term, you cannot as easily adapt the weighted sum of inputs (i.e. mx) to fit the data (i.e. in the example of a simple line, the line cannot move up and down the y-axis without that b term).
w in the diagram above stands for the weights, and x stands for the input values.
Here is a similar diagram, but now it is a two-layer neural network instead of single layer.
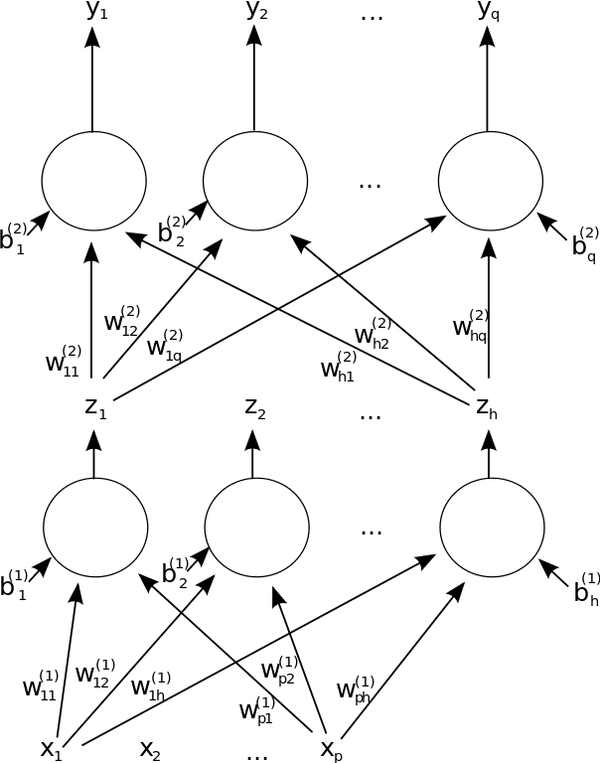
And here is one last way to look at the same thing I explained above:
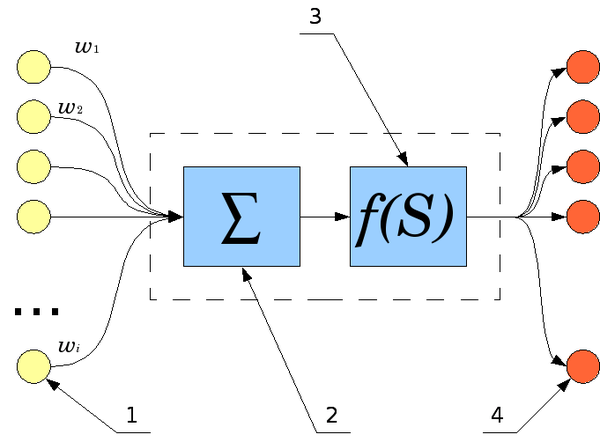
Note that the yellow circles on the left represent the input values. w represents the weights. The sigma inside the box means that we calculated the weighted sum of the input values. We run that through the activation function f(S)…e.g. sigmoid function. And then out of that, pops the output, which is passed on to the nodes in the following layer.
Neural networks that contain many layers, for example more than 100, are called deep neural networks. Deep neural networks are the cornerstone of the rapidly growing field known as deep learning.
Training Phase
The objective during the training phase of a neural network is to determine all the connection weights. At the start of training, the weights of the network are initialized to small random values close to 0. After this step, training proceeds to the two main phases of the algorithm: forward propagation and backpropagation.
Forward Propagation
During the forward propagation phase of a neural network, we process one instance (i.e. one set of inputs) at a time. Hidden layers extract important features contained in the input data by computing a weighted sum of the inputs and running the result through the logistic sigmoid activation function. This output relays to nodes in the next hidden layer where the data is transformed yet again. This process continues until the data reaches the output layer.
The output of the output layer is a predicted class value, which in this project is a one-hot encoded class prediction vector. The index of the vector corresponds to each class. For example, if a 1 is in the 0 index of the vector (and a 0 is in all other indices of the vector), the class prediction is class 0. Because we are dealing with 0s and 1s, the output vector can also be considered the probability that an instance is in a given class.
Backpropagation
After the input signal produced by a training instance propagates through the network one layer at a time to the output layer, the backpropagation phase commences. An error value is calculated at the output layer. This error corresponds to the difference between the class predicted by the network and the actual (i.e. true) class of the training instance.
The prediction error is then propagated backward from the output layer to the input layer. Blame for the error is assigned to each node in each layer, and then the weights of each node of the neural network are updated accordingly (with the goal to make more accurate class predictions for the next instance that flows through the neural network) using stochastic gradient descent for the weight optimization procedure.
Note that weights of the neural network are adjusted on a training instance by training instance basis. This online learning method is the preferred one for classification problems of large size (Ĭordanov & Jain, 2013).
The forward propagation and backpropagation phases continue for a certain number of epochs. A single epoch finishes when each training instance has been processed exactly once.
Testing Phase
Once the neural network has been trained, it can be used to make predictions on new, unseen test instances. Test instances flow through the network one-by-one, and the resulting output (which is a vector of class probabilities) determines the classification.
Helpful Video
Below is a helpful video by Andrew Ng, a professor at Stanford University, that explains neural networks and is helpful for getting your head around the math. The video gets pretty complicated in some spots (esp. where he starts writing all sorts of mathematical notation and derivatives). My advice is to lookup anything that he explains that isn’t clear. Take it slow as you are learning about neural networks. There is no rush. This stuff isn’t easy to understand on your first encounter with it. Over time, the fog will begin to lift, and you will be able to understand how it all works.
Artificial Feedforward Neural Network Trained with Backpropagation Algorithm Design
The Logistic Regression algorithm was implemented from scratch. The Breast Cancer, Glass, Iris, Soybean (small), and Vote data sets were preprocessed to meet the input requirements of the algorithms. I used five-fold stratified cross-validation to evaluate the performance of the models.
Required Data Set Format for Feedforward Neural Network Trained with Backpropagation
Columns (0 through N)
- 0: Instance ID
- 1: Attribute 1
- 2: Attribute 2
- 3: Attribute 3
- …
- N: Actual Class
The program then adds two additional columns for the testing set.
- N + 1: Predicted Class
- N + 2: Prediction Correct? (1 if yes, 0 if no)
Breast Cancer Data Set
This breast cancer data set contains 699 instances, 10 attributes, and a class – malignant or benign (Wolberg, 1992).
Modification of Attribute Values
- The actual class value was changed to “Benign” or “Malignant.”
- Attribute values were normalized to be in the range 0 to 1.
- Class values were vectorized using one-hot encoding.
Missing Data
There were 16 missing attribute values, each denoted with a “?”. I chose a random number between 1 and 10 (inclusive) to fill in the data.
Glass Data Set
This glass data set contains 214 instances, 10 attributes, and 7 classes (German, 1987). The purpose of the data set is to identify the type of glass.
Modification of Attribute Values
- Attribute values were normalized to be in the range 0 to 1.
- Class values were vectorized using one-hot encoding.
Missing Data
There are no missing values in this data set.
Iris Data Set
This data set contains 3 classes of 50 instances each (150 instances in total), where each class refers to a different type of iris plant (Fisher, 1988).
Modification of Attribute Values
- Attribute values were normalized to be in the range 0 to 1.
- Class values were vectorized using one-hot encoding.
Missing Data
There were no missing attribute values.
Soybean Data Set (small)
This soybean (small) data set contains 47 instances, 35 attributes, and 4 classes (Michalski, 1980). The purpose of the data set is to determine the disease type.
Modification of Attribute Values
- Attribute values were normalized to be in the range 0 to 1.
- Class values were vectorized using one-hot encoding.
- Attribute values that were all the same value were removed.
Missing Data
There are no missing values in this data set.
Vote Data Set
This data set includes votes for each of the U.S. House of Representatives Congressmen (435 instances) on the 16 key votes identified by the Congressional Quarterly Almanac (Schlimmer, 1987). The purpose of the data set is to identify the representative as either a Democrat or Republican.
- 267 Democrats
- 168 Republicans
Modification of Attribute Values
- I did the following modifications:
- Changed all “y” to 1 and all “n” to 0.
- Class values were vectorized using one-hot encoding.
Missing Data
Missing values were denoted as “?”. To fill in those missing values, I chose random number, either 0 (“No”) or 1 (“Yes”).
Stochastic Gradient Descent
I used stochastic gradient descent for optimizing the weights.
In normal gradient descent, we need to calculate the partial derivative of the cost function with respect to each weight. For each partial derivative, we have to tally up the terms for each training instance to compute the partial derivative of the cost function with respect to that weight. What this means is that, if we have a lot of attributes and a large dataset, gradient descent is slow. For this reason, stochastic gradient descent was chosen since weights are updated after each training instance (as opposed to after all training instances).
Here is a good video that explains stochastic gradient descent.
Logistic (Sigmoid) Activation Function
The logistic (sigmoid) activation function was used for the nodes in the neural network.
Description of Any Tuning Process Applied
Learning Rate
Some tuning was performed in this project. The learning rate was set to 0.1, which was different than the 0.01 value that is often used for multi-layer feedforward neural networks (Montavon, 2012). Lower values resulted in much longer training times and did not result in large improvements in classification accuracy.
Epochs
The number of epochs chosen for the main runs of the algorithm on the data sets was chosen to be 1000. Other values were tested, but the number of epochs did not have a large impact on classification accuracy.
Number of Nodes per Hidden Layer
In order to tune the number of nodes per hidden layer, I used a constant learning rate and constant number of epochs. I then calculated the classification accuracy for each data set for a set number of nodes per hidden layer. I performed this process using networks with one hidden layer and networks with two hidden layers. The results of this tuning process are below.
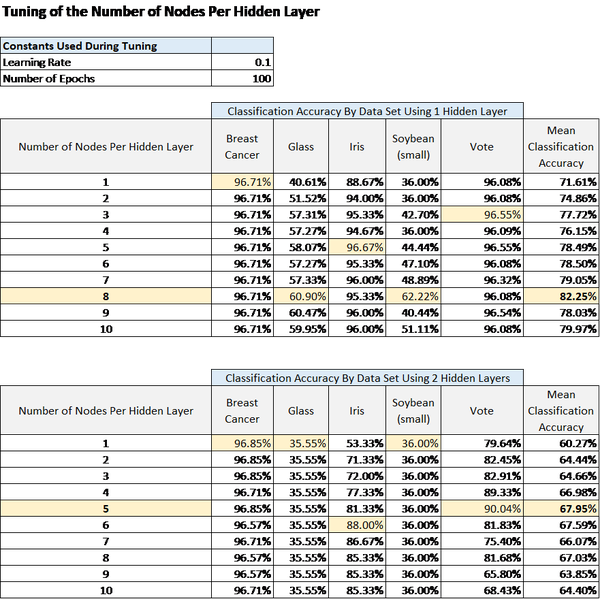
Note that the mean classification accuracy across all data sets when one hidden layer was used for the neural network reached a peak at eight nodes per hidden layer. This value of eight nodes per hidden layer was used for the actual runs on the data sets.
For two hidden layers, the peak mean classification accuracy was attained at five nodes per hidden layer. Thus, when the algorithm was run on the data sets for two hidden layers, I used five nodes per hidden layer for each data set to compare the classification accuracy across the data sets.
Artificial Feedforward Neural Network Trained with Backpropagation Algorithm in Python, Coded From Scratch
Here are the preprocessed data sets:
Here is the full code for the neural network. This is all you need to run the program:
import pandas as pd # Import Pandas library
import numpy as np # Import Numpy library
from random import shuffle # Import shuffle() method from the random module
from random import seed # Import seed() method from the random module
from random import random # Import random() method from the random module
from collections import Counter # Used for counting
from math import exp # Import exp() function from the math module
# File name: neural_network.py
# Author: Addison Sears-Collins
# Date created: 7/30/2019
# Python version: 3.7
# Description: An artificial feedforward neural network trained
# with backpropagation (also called multilayer perceptron)
# Required Data Set Format
# Columns (0 through N)
# 0: Attribute 0
# 1: Attribute 1
# 2: Attribute 2
# 3: Attribute 3
# ...
# N: Actual Class
# 2 additional columns are added for the test set.
# N + 1: Predicted Class
# N + 2: Prediction Correct?
ALGORITHM_NAME = "Feedforward Neural Network With Backpropagation"
SEPARATOR = "," # Separator for the data set (e.g. "\t" for tab data)
def normalize(dataset):
"""
Normalize the attribute values so that they are between 0 and 1, inclusive
:param pandas_dataframe dataset: The original dataset as a Pandas dataframe
:return: normalized_dataset
:rtype: Pandas dataframe
"""
# Generate a list of the column names
column_names = list(dataset)
# For every column except the actual class column
for col in range(0, len(column_names) - 1):
temp = dataset[column_names[col]] # Go column by column
minimum = temp.min() # Get the minimum of the column
maximum = temp.max() # Get the maximum of the column
# Normalized all values in the column so that they
# are between 0 and 1.
# x_norm = (x_i - min(x))/(max(x) - min(x))
dataset[column_names[col]] = dataset[column_names[col]] - minimum
dataset[column_names[col]] = dataset[column_names[col]] / (
maximum - minimum)
normalized_dataset = dataset
return normalized_dataset
def get_five_stratified_folds(dataset):
"""
Implementation of five-fold stratified cross-validation. Divide the data
set into five random folds. Make sure that the proportion of each class
in each fold is roughly equal to its proportion in the entire data set.
:param pandas_dataframe dataset: The original dataset as a Pandas dataframe
:return: five_folds
:rtype: list of folds where each fold is a list of instances(i.e. examples)
"""
# Create five empty folds
five_folds = list()
fold0 = list()
fold1 = list()
fold2 = list()
fold3 = list()
fold4 = list()
# Get the number of columns in the data set
class_column = len(dataset[0]) - 1
# Shuffle the data randomly
shuffle(dataset)
# Generate a list of the unique class values and their counts
classes = list() # Create an empty list named 'classes'
# For each instance in the dataset, append the value of the class
# to the end of the classes list
for instance in dataset:
classes.append(instance[class_column])
# Create a list of the unique classes
unique_classes = list(Counter(classes).keys())
# For each unique class in the unique class list
for uniqueclass in unique_classes:
# Initialize the counter to 0
counter = 0
# Go through each instance of the data set and find instances that
# are part of this unique class. Distribute them among one
# of five folds
for instance in dataset:
# If we have a match
if uniqueclass == instance[class_column]:
# Allocate instance to fold0
if counter == 0:
# Append this instance to the fold
fold0.append(instance)
# Increase the counter by 1
counter += 1
# Allocate instance to fold1
elif counter == 1:
# Append this instance to the fold
fold1.append(instance)
# Increase the counter by 1
counter += 1
# Allocate instance to fold2
elif counter == 2:
# Append this instance to the fold
fold2.append(instance)
# Increase the counter by 1
counter += 1
# Allocate instance to fold3
elif counter == 3:
# Append this instance to the fold
fold3.append(instance)
# Increase the counter by 1
counter += 1
# Allocate instance to fold4
else:
# Append this instance to the fold
fold4.append(instance)
# Reset the counter to 0
counter = 0
# Shuffle the folds
shuffle(fold0)
shuffle(fold1)
shuffle(fold2)
shuffle(fold3)
shuffle(fold4)
# Add the five stratified folds to the list
five_folds.append(fold0)
five_folds.append(fold1)
five_folds.append(fold2)
five_folds.append(fold3)
five_folds.append(fold4)
return five_folds
def initialize_neural_net(
no_inputs, no_hidden_layers, no_nodes_per_hidden_layer, no_outputs):
"""
Generates a new neural network that is ready to be trained.
Network (list of layers): 0+ hidden layers, and output layer
Input Layer (list of attribute values): A row from the training set
Hidden Layer (list of dictionaries): A set of nodes (i.e. neurons)
Output Layer (list of dictionaries): A set of nodes, one node per class
Node (dictionary): Contains a set of weights, one weight for each input
to the layer containing that node + an additional weight for the bias.
Each node is represented as a dictionary that stores key-value pairs
Each key corresponds to a property of that node (e.g. weights).
Weights will be initialized to small random values between 0 and 1.
:param int no_inputs: Numper of inputs (i.e. attributes)
:param int no_hidden_layers: Numper of hidden layers (0 or more)
:param int no_nodes_per_hidden_layer: Numper of nodes per hidden layer
:param int no_outputs: Numper of outputs (one output node per class)
:return: network
:rtype:list (i.e. list of layers: hidden layers, output layer)
"""
# Create an empty list
network = list()
# Create the the hidden layers
hidden_layer = list()
hl_counter = 0
# Create the output layer
output_layer = list()
# If this neural network contains hidden layers
if no_hidden_layers > 0:
# Build one hidden layer at a time
for layer in range(no_hidden_layers):
# Reset to an empty hidden layer
hidden_layer = list()
# If this is the first hidden layer
if hl_counter == 0:
# Build one node at a time
for node in range(no_nodes_per_hidden_layer):
initial_weights = list()
# Each node in the hidden layer has no_inputs + 1 weights,
# initialized to a random number in the range [0.0, 1.0)
for i in range(no_inputs + 1):
initial_weights.append(random())
# Add the node to the first hidden layer
hidden_layer.append({'weights':initial_weights})
# Finished building the first hidden layer
hl_counter += 1
# Add this first hidden layer to the front of the neural
# network
network.append(hidden_layer)
# If this is not the first hidden layer
else:
# Build one node at a time
for node in range(no_nodes_per_hidden_layer):
initial_weights = list()
# Each node in the hidden layer has
# no_nodes_per_hidden_layer + 1 weights, initialized to
# a random number in the range [0.0, 1.0)
for i in range(no_nodes_per_hidden_layer + 1):
initial_weights.append(random())
hidden_layer.append({'weights':initial_weights})
# Add this newly built hidden layer to the neural network
network.append(hidden_layer)
# Build the output layer
for outputnode in range(no_outputs):
initial_weights = list()
# Each node in the output layer has no_nodes_per_hidden_layer
# + 1 weights, initialized to a random number in
# the range [0.0, 1.0)
for i in range(no_nodes_per_hidden_layer + 1):
initial_weights.append(random())
# Add this output node to the output layer
output_layer.append({'weights':initial_weights})
# Add the output layer to the neural network
network.append(output_layer)
# A neural network has no hidden layers
else:
# Build the output layer
for outputnode in range(no_outputs):
initial_weights = list()
# Each node in the hidden layer has no_inputs + 1 weights,
# initialized to a random number in the range [0.0, 1.0)
for i in range(no_inputs + 1):
initial_weights.append(random())
# Add this output node to the output layer
output_layer.append({'weights':initial_weights})
network.append(output_layer)
# Finished building the initial neural network
return network
def weighted_sum_of_inputs(weights, inputs):
"""
Calculates the weighted sum of inputs plus the bias
:param list weights: A list of weights. Each node has a list of weights.
:param list inputs: A list of input values. These can be a single row
of attribute values or the output from nodes from the previous layer
:return: weighted_sum
:rtype: float
"""
# We assume that the last weight is the bias value
# The bias value is a special weight that does not multiply with an input
# value (or we could assume its corresponding input value is always 1)
# The bias is similar to the intercept constant b in y = mx + b. It enables
# a (e.g. sigmoid) curve to be shifted to create a better fit
# to the data. Without the bias term b, the line always goes through the
# origin (0,0) and cannot adapt as well to the data.
# In y = mx + b, we assume b * x_0 where x_0 = 1
# Initiate the weighted sum with the bias term. Assume the last weight is
# the bias term
weighted_sum = weights[-1]
for index in range(len(weights) - 1):
weighted_sum += weights[index] * inputs[index]
return weighted_sum
def sigmoid(weighted_sum_of_inputs_plus_bias):
"""
Run the weighted sum of the inputs + bias through
the sigmoid activation function.
:param float weighted_sum_of_inputs_plus_bias: Node summation term
:return: sigmoid(weighted_sum_of_inputs_plus_bias)
"""
return 1.0 / (1.0 + exp(-weighted_sum_of_inputs_plus_bias))
def forward_propagate(network, instance):
"""
Instances move forward through the neural network from one layer
to the next layer. At each layer, the outputs are calculated for each
node. These outputs are the inputs for the nodes in the next layer.
The last set of outputs is the output for the nodes in the output
layer.
:param list network: List of layers: 0+ hidden layers, 1 output layer
:param list instance (a single training/test instance from the data set)
:return: outputs
:rtype: list
"""
inputs = instance
# For each layer in the neural network
for layer in network:
# These will store the outputs for this layer
new_inputs = list()
# For each node in this layer
for node in layer:
# Calculate the weighted sum + bias term
weighted_sum = weighted_sum_of_inputs(node['weights'], inputs)
# Run the weighted sum through the activation function
# and store the result in this node's dictionary.
# Now the node's dictionary has two keys, weights and output.
node['output'] = sigmoid(weighted_sum)
# Used for debugging
#print(node)
# Add the output of the node to the new_inputs list
new_inputs.append(node['output'])
# Update the inputs list
inputs = new_inputs
# We have reached the output layer
outputs = inputs
return outputs
def sigmoid_derivative(output):
"""
The derivative of the sigmoid activation function with respect
to the weighted summation term of the node.
Formally (after a lot of calculus), this derivative is:
derivative = sigmoid(weighted_sum_of_inputs_plus_bias) *
(1 - sigmoid(weighted_sum_of_inputs_plus_bias))
= node_ouput * (1 - node_output)
This method is used during the backpropagation phase.
:param list output: Output of a node (generated during the forward
propagation phase)
:return: sigmoid_der
:rtype: float
"""
return output * (1.0 - output)
def back_propagate(network, actual):
"""
In backpropagation, the error is computed between the predicted output by
the network and the actual output as determined by the data set. This error
propagates backwards from the output layer to the first hidden layer. The
weights in each layer are updated along the way in response to the error.
The goal is to reduce the prediction error for the next training instance
that forward propagates through the network.
:param network list: The neural network
:param actual list: A list of the actual output from the data set
"""
# Iterate in reverse order (i.e. starts from the output layer)
for i in reversed(range(len(network))):
# Work one layer at a time
layer = network[i]
# Keep track of the errors for the nodes in this layer
errors = list()
# If this is a hidden layer
if i != len(network) - 1:
# For each node_j in this hidden layer
for j in range(len(layer)):
# Reset the error value
error = 0.0
# Calculate the weighted error.
# The error values come from the error (i.e. delta) calculated
# at each node in the layer just to the "right" of this layer.
# This error is weighted by the weight connections between the
# node in this hidden layer and the nodes in the layer just
# to the "right" of this layer.
for node in network[i + 1]:
error += (node['weights'][j] * node['delta'])
# Add the weighted error for node_j to the
# errors list
errors.append(error)
# If this is the output layer
else:
# For each node in the output layer
for j in range(len(layer)):
# Store this node (i.e. dictionary)
node = layer[j]
# Actual - Predicted = Error
errors.append(actual[j] - node['output'])
# Calculate the delta for each node_j in this layer
for j in range(len(layer)):
node = layer[j]
# Add an item to the node's dictionary with the
# key as delta.
node['delta'] = errors[j] * sigmoid_derivative(node['output'])
def update_weights(network, instance, learning_rate):
"""
After the deltas (errors) have been calculated for each node in
each layer of the neural network, the weights can be updated.
new_weight = old_weight + learning_rate * delta * input_value
:param list network: List of layers: 0+ hidden layers, 1 output layer
:param list instance: A single training/test instance from the data set
:param float learning_rate: Controls step size in the stochastic gradient
descent procedure.
"""
# For each layer in the network
for layer_index in range(len(network)):
# Extract all the attribute values, excluding the class value
inputs = instance[:-1]
# If this is not the first hidden layer
if layer_index != 0:
# Go through each node in the previous layer and add extract the
# output from that node. The output from the previous layer
# is the input to this layer.
inputs = [node['output'] for node in network[layer_index - 1]]
# For each node in this layer
for node in network[layer_index]:
# Go through each input value
for j in range(len(inputs)):
# Update the weights
node['weights'][j] += learning_rate * node['delta'] * inputs[j]
# Updating the bias weight
node['weights'][-1] += learning_rate * node['delta']
def train_neural_net(
network, training_set, learning_rate, no_epochs, no_outputs):
"""
Train a neural network that has already been initialized.
Training is done using stochastic gradient descent where the weights
are updated one training instance at a time rather than after the
entire training set (as is the case with gradient descent).
:param list network: The neural network, which is a list of layers
:param list training_set: A list of training instances (i.e. examples)
:param float learning_rate: Controls step size of gradient descent
:param int no_epochs: How many passes we will make through training set
:param int no_outputs: The number of output nodes (equal to # of classes)
"""
# Go through the entire training set a fixed number of times (i.e. epochs)
for epoch in range(no_epochs):
# Update the weights one instance at a time
for instance in training_set:
# Forward propagate the training instance through the network
# and produce the output, which is a list.
outputs = forward_propagate(network, instance)
# Vectorize the output using one hot encoding.
# Create a list called actual_output that is the same length
# as the number of outputs. Put a 1 in the place of the actual
# class.
actual_output = [0 for i in range(no_outputs)]
actual_output[int(instance[-1])] = 1
back_propagate(network, actual_output)
update_weights(network, instance, learning_rate)
def predict_class(network, instance):
"""
Make a class prediction given a trained neural network and
an instance from the test data set.
:param list network: The neural network, which is a list of layers
:param list instance: A single training/test instance from the data set
:return class_prediction
:rtype int
"""
outputs = forward_propagate(network, instance)
# Return the index that has the highest probability. This index
# is the class value. Assume class values begin at 0 and go
# upwards by 1 (i.e. 0, 1, 2, ...)
class_prediction = outputs.index(max(outputs))
return class_prediction
def calculate_accuracy(actual, predicted):
"""
Calculates the accuracy percentages
:param list actual: Actual class values
:param list predicted: predicted class values
:return: classification_accuracy
:rtype: float (as a percentage)
"""
number_of_correct_predictions = 0
for index in range(len(actual)):
if actual[index] == predicted[index]:
number_of_correct_predictions += 1
classification_accuracy = (
number_of_correct_predictions / float(len(actual))) * 100.0
return classification_accuracy
def get_test_set_predictions(
training_set, test_set, learning_rate, no_epochs,
no_hidden_layers, no_nodes_per_hidden_layer):
"""
This method is the workhorse.
A new neutal network is initialized.
The network is trained on the training set.
The trained neural network is used to generate predictions on the
test data set.
:param list training_set
:param list test_set
:param float learning_rate
:param int no_epochs
:param int no_hidden_layers
:param int no_nodes_per_hidden_layer
:return network, class_predictions
:rtype list, list
"""
# Get the number of attribute values
no_inputs = len(training_set[0]) - 1
# Calculate the number of unique classes
no_outputs = len(set([instance[-1] for instance in training_set]))
# Build a new neural network
network = initialize_neural_net(
no_inputs, no_hidden_layers, no_nodes_per_hidden_layer, no_outputs)
train_neural_net(
network, training_set, learning_rate, no_epochs, no_outputs)
# Store the class predictions for each test instance
class_predictions = list()
for instance in test_set:
cl_prediction = predict_class(network, instance)
class_predictions.append(cl_prediction)
# Return the learned model as well as the class predictions
return network, class_predictions
###############################################################
def main():
"""
The main method of the program
"""
LEARNING_RATE = 0.1 # Used for stochastic gradient descent procedure
NO_EPOCHS = 1000 # Epoch is one complete pass through training data
NO_HIDDEN_LAYERS = 1 # Number of hidden layers
NO_NODES_PER_HIDDEN_LAYER = 8 # Number of nodes per hidden layer
# Welcome message
print("Welcome to the " + ALGORITHM_NAME + " Program!")
print()
# Directory where data set is located
#data_path = input("Enter the path to your input file: ")
data_path = "vote.txt"
# Read the full text file and store records in a Pandas dataframe
pd_data_set = pd.read_csv(data_path, sep=SEPARATOR)
# Show functioning of the program
#trace_runs_file = input("Enter the name of your trace runs file: ")
trace_runs_file = "vote_nn_trace_runs.txt"
## Open a new file to save trace runs
outfile_tr = open(trace_runs_file,"w")
# Testing statistics
#test_stats_file = input("Enter the name of your test statistics file: ")
test_stats_file = "vote_nn_test_stats.txt"
## Open a test_stats_file
outfile_ts = open(test_stats_file,"w")
# Generate a list of the column names
column_names = list(pd_data_set)
# The input layer in the neural network
# will have one node for each attribute value
no_of_inputs = len(column_names) - 1
# Make a list of the unique classes
list_of_unique_classes = pd.unique(pd_data_set["Actual Class"])
# The output layer in the neural network
# will have one node for each class value
no_of_outputs = len(list_of_unique_classes)
# Replace all the class values with numbers, starting from 0
# in the Pandas dataframe.
for cl in range(0, len(list_of_unique_classes)):
pd_data_set["Actual Class"].replace(
list_of_unique_classes[cl], cl ,inplace=True)
# Normalize the attribute values so that they are all between 0
# and 1, inclusive
normalized_pd_data_set = normalize(pd_data_set)
# Convert normalized Pandas dataframe into a list
dataset_as_list = normalized_pd_data_set.values.tolist()
# Set the seed for random number generator
seed(1)
# Get a list of 5 stratified folds because we are doing
# five-fold stratified cross-validation
fv_folds = get_five_stratified_folds(dataset_as_list)
# Keep track of the scores for each of the five experiments
scores = list()
experiment_counter = 0
for fold in fv_folds:
print()
print("Running Experiment " + str(experiment_counter) + " ...")
print()
outfile_tr.write("Running Experiment " + str(
experiment_counter) + " ...\n")
outfile_tr.write("\n")
# Get all the folds and store them in the training set
training_set = list(fv_folds)
# Four folds make up the training set
training_set.remove(fold)
# Combined all the folds so that all we have is a list
# of training instances
training_set = sum(training_set, [])
# Initialize a test set
test_set = list()
# For each instance in this test fold
for instance in fold:
# Create a copy and store it
copy_of_instance = list(instance)
test_set.append(copy_of_instance)
# Get the trained neural network and the predicted values
# for each test instance
neural_net, predicted_values = get_test_set_predictions(
training_set, test_set,LEARNING_RATE,NO_EPOCHS,
NO_HIDDEN_LAYERS,NO_NODES_PER_HIDDEN_LAYER)
actual_values = [instance[-1] for instance in fold]
accuracy = calculate_accuracy(actual_values, predicted_values)
scores.append(accuracy)
# Print the learned model
print("Experiment " + str(
experiment_counter) + " Trained Neural Network")
print()
for layer in neural_net:
print(layer)
print()
outfile_tr.write("Experiment " + str(
experiment_counter) + " Trained Neural Network")
outfile_tr.write("\n")
outfile_tr.write("\n")
for layer in neural_net:
outfile_tr.write(str(layer))
outfile_tr.write("\n")
outfile_tr.write("\n\n")
# Print the classifications on the test instances
print("Experiment " + str(
experiment_counter) + " Classifications on Test Instances")
print()
outfile_tr.write("Experiment " + str(
experiment_counter) + " Classifications on Test Instances")
outfile_tr.write("\n\n")
test_df = pd.DataFrame(test_set, columns=column_names)
# Add 2 additional columns to the testing dataframe
test_df = test_df.reindex(
columns=[*test_df.columns.tolist(
), 'Predicted Class', 'Prediction Correct?'])
# Add the predicted values to the "Predicted Class" column
# Indicate if the prediction was correct or not.
for pre_val_index in range(len(predicted_values)):
test_df.loc[pre_val_index, "Predicted Class"] = predicted_values[
pre_val_index]
if test_df.loc[pre_val_index, "Actual Class"] == test_df.loc[
pre_val_index, "Predicted Class"]:
test_df.loc[pre_val_index, "Prediction Correct?"] = "Yes"
else:
test_df.loc[pre_val_index, "Prediction Correct?"] = "No"
# Replace all the class values with the name of the class
for cl in range(0, len(list_of_unique_classes)):
test_df["Actual Class"].replace(
cl, list_of_unique_classes[cl] ,inplace=True)
test_df["Predicted Class"].replace(
cl, list_of_unique_classes[cl] ,inplace=True)
# Print out the test data frame
print(test_df)
print()
print()
outfile_tr.write(str(test_df))
outfile_tr.write("\n\n")
# Go to the next experiment
experiment_counter += 1
print("Experiments Completed.\n")
outfile_tr.write("Experiments Completed.\n\n")
# Print the test stats
print("------------------------------------------------------------------")
print(ALGORITHM_NAME + " Summary Statistics")
print("------------------------------------------------------------------")
print("Data Set : " + data_path)
print()
print("Learning Rate: " + str(LEARNING_RATE))
print("Number of Epochs: " + str(NO_EPOCHS))
print("Number of Hidden Layers: " + str(NO_HIDDEN_LAYERS))
print("Number of Nodes Per Hidden Layer: " + str(
NO_NODES_PER_HIDDEN_LAYER))
print()
print("Accuracy Statistics for All 5 Experiments: %s" % scores)
print()
print()
print("Classification Accuracy: %.3f%%" % (
sum(scores)/float(len(scores))))
outfile_ts.write(
"------------------------------------------------------------------\n")
outfile_ts.write(ALGORITHM_NAME + " Summary Statistics\n")
outfile_ts.write(
"------------------------------------------------------------------\n")
outfile_ts.write("Data Set : " + data_path +"\n\n")
outfile_ts.write("Learning Rate: " + str(LEARNING_RATE) + "\n")
outfile_ts.write("Number of Epochs: " + str(NO_EPOCHS) + "\n")
outfile_ts.write("Number of Hidden Layers: " + str(
NO_HIDDEN_LAYERS) + "\n")
outfile_ts.write("Number of Nodes Per Hidden Layer: " + str(
NO_NODES_PER_HIDDEN_LAYER) + "\n")
outfile_ts.write(
"Accuracy Statistics for All 5 Experiments: %s" % str(scores))
outfile_ts.write("\n\n")
outfile_ts.write("Classification Accuracy: %.3f%%" % (
sum(scores)/float(len(scores))))
## Close the files
outfile_tr.close()
outfile_ts.close()
main()
Artificial Feedforward Neural Network Trained with Backpropagation Output
Here are the trace runs:
- Breast Cancer
- Glass
- Iris
- Soybean (small)
- Vote
Here are the results:
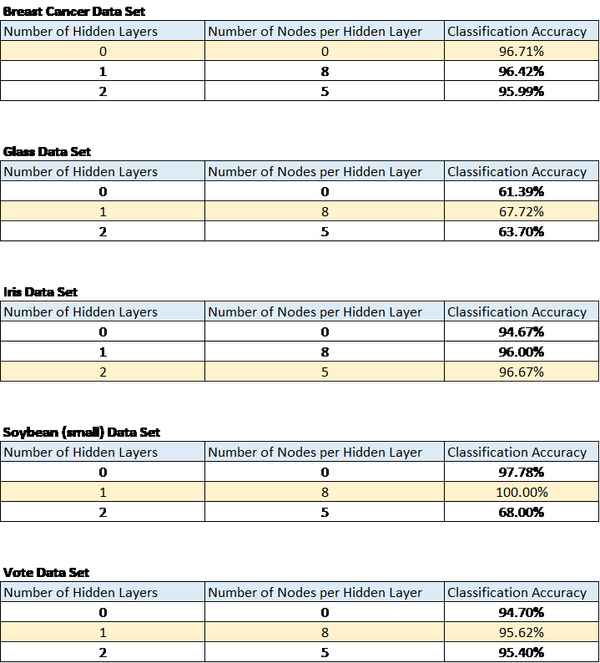
Here are the test statistics for each data set:
- Breast Cancer
- Glass
- Iris
- Soybean (small)
- Vote
Analysis
Breast Cancer Data Set
The breast cancer data set results were in line with what I expected. The simpler model, the one with no hidden layers, ended up generating the highest classification accuracy. Classification accuracy was just short of 97%. In other words, the neural network that had no hidden layers successfully classified a patient as either malignant or benign with an almost 97% accuracy.
These results also suggest that the amount of training data has a direct impact on performance. Higher amounts of data (699 instances in this case) can lead to better learning and better classification accuracy on new, unseen instances.
Glass Data Set
The performance of the neural network on the glass data set was the worst out of all of the data sets. The ability of the network to correctly identify the type of glass given the attribute values never exceeded 70%.
I hypothesize that the poor performance on the glass data set is due to the high numbers of classes combined with a relatively smaller data set.
Iris Data Set
Classification accuracy was superb on the iris dataset, attaining a classification accuracy around 97%. The results of the iris dataset were surprising given that the more complicated neural network with two hidden layers and five nodes per hidden layer outperformed the simpler neural network that had no hidden layers. In this case, it appears that the iris dataset benefited from the increasing layers of abstraction provided by a higher number of layers.
Soybean Data Set (small)
Performance on the soybean data set was stellar and was the highest of all of the data sets but also had the largest standard deviation for the classification accuracy. Note that classification accuracy reached a peak of 100% using one hidden layer and eight nodes for the hidden layer. However, when I added an additional hidden layer, classification accuracy dropped to under 70%.
The reason for the high standard deviation of the classification accuracy is unclear, but I hypothesize it has to do with the relatively small number of training instances. Future work would need to be performed with the soybean large dataset available from the UCI Machine Learning Repository to see if these results remain consistent.
The results of the soybean runs suggest that large numbers of relevant attributes can help a machine learning algorithm create more accurate classifications.
Vote Data Set
The vote data set did not yield the stellar performance of the soybean data set, but classification accuracy was still solid at ~96% using one hidden layer and eight nodes per hidden layer. These results are in line with what I expected because voting behavior should provide a powerful predictor of whether a candidate is a Democrat or Republican. I would have been surprised had I observed classification accuracies that were lower since members of Congress tend to vote along party lines on most issues.
Summary and Conclusions
My hypothesis was incorrect. In some cases, simple neural networks with no hidden layers outperformed more complex neural networks with 1+ hidden layers. However, in other cases, more complex neural networks with multiple hidden layers outperformed the network with no hidden layers. The reason why some data is more amenable to networks with hidden layers instead of without hidden layers is unclear.
Other conclusions include the following:
- Higher amounts of data can lead to better learning and better classification accuracy on new, unseen instances.
- Large numbers of relevant attributes can help a neural network create more accurate classifications.
- Neural networks are powerful and can achieve excellent results on both binary and multi-class classification problems.
References
Alpaydin, E. (2014). Introduction to Machine Learning. Cambridge, Massachusetts: The MIT Press.
Fisher, R. (1988, July 01). Iris Data Set. Retrieved from Machine Learning Repository: https://archive.ics.uci.edu/ml/datasets/iris
German, B. (1987, September 1). Glass Identification Data Set. Retrieved from UCI Machine Learning Repository: https://archive.ics.uci.edu/ml/datasets/Glass+Identification
Ĭordanov, I., & Jain, L. C. (2013). Innovations in Intelligent Machines –3 : Contemporary Achievements in Intelligent Systems. Berlin: Springer.
Michalski, R. (1980). Learning by being told and learning from examples: an experimental comparison of the two methodes of knowledge acquisition in the context of developing an expert system for soybean disease diagnosis. International Journal of Policy Analysis and Information Systems, 4(2), 125-161.
Montavon, G. O. (2012). Neural Networks : Tricks of the Trade. New York: Springer.
Schlimmer, J. (1987, 04 27). Congressional Voting Records Data Set. Retrieved from Machine Learning Repository: https://archive.ics.uci.edu/ml/datasets/Congressional+Voting+Records
Wolberg, W. (1992, 07 15). Breast Cancer Wisconsin (Original) Data Set. Retrieved from Machine Learning Repository: https://archive.ics.uci.edu/ml/datasets/Breast+Cancer+Wisconsin+%28Original%25