In this tutorial, we will install OpenCV 4.5 on the NVIDIA Jetson Nano. The reason I will install OpenCV 4.5 is because the OpenCV that comes pre-installed on the Jetson Nano does not have CUDA support. CUDA support will enable us to use the GPU to run deep learning applications.
The terminal command to check which OpenCV version you have on your computer is:
python -c 'import cv2; print(cv2.__version__)'
Prerequisites
- You have already set up your NVIDIA Jetson Nano (4GB, B01).
- If you didn’t follow my setup guide in the bullet point above, make sure you create a Swap file. See the “Create a Swap File” section of this tutorial on how to do that.
Install Dependencies
Type the following command.
sudo sh -c "echo '/usr/local/cuda/lib64' >> /etc/ld.so.conf.d/nvidia-tegra.conf"
Create the links and caching to the shared libraries
sudo ldconfig
Install the relevant third party libraries. Play close attention to the line wrapping below. Each command begins with “sudo apt-get install”.
sudo apt-get install build-essential cmake git unzip pkg-config
sudo apt-get install libjpeg-dev libpng-dev libtiff-dev
sudo apt-get install libavcodec-dev libavformat-dev libswscale-dev
sudo apt-get install libgtk2.0-dev libcanberra-gtk*
sudo apt-get install python3-dev python3-numpy python3-pip
sudo apt-get install libxvidcore-dev libx264-dev libgtk-3-dev
sudo apt-get install libtbb2 libtbb-dev libdc1394-22-dev
sudo apt-get install libv4l-dev v4l-utils
sudo apt-get install libgstreamer1.0-dev libgstreamer-plugins-base1.0-dev
sudo apt-get install libavresample-dev libvorbis-dev libxine2-dev
sudo apt-get install libfaac-dev libmp3lame-dev libtheora-dev
sudo apt-get install libopencore-amrnb-dev libopencore-amrwb-dev
sudo apt-get install libopenblas-dev libatlas-base-dev libblas-dev
sudo apt-get install liblapack-dev libeigen3-dev gfortran
sudo apt-get install libhdf5-dev protobuf-compiler
sudo apt-get install libprotobuf-dev libgoogle-glog-dev libgflags-dev
Download OpenCV
Now that we’ve installed the third-party libraries, let’s install OpenCV itself. The latest release is listed here. Again, pay attention to the line wrapping. The https://github… was too long to fit on one line.
cd ~
wget -O opencv.zip https://github.com/opencv/opencv/archive/4.5.1.zip
wget -O opencv_contrib.zip https://github.com/opencv/opencv_contrib/archive/4.5.1.zip
unzip opencv.zip
unzip opencv_contrib.zip
Now rename the directories. Type each command below, one after the other.
mv opencv-4.5.1 opencv
mv opencv_contrib-4.5.1 opencv_contrib
rm opencv.zip
rm opencv_contrib.zip
Build OpenCV
Let’s build the OpenCV files.
Create a directory.
cd ~/opencv
mkdir build
cd build
Set the compilation directives. You can copy and paste this entire block of commands below into your terminal.
cmake -D CMAKE_BUILD_TYPE=RELEASE \
-D CMAKE_INSTALL_PREFIX=/usr \
-D OPENCV_EXTRA_MODULES_PATH=~/opencv_contrib/modules \
-D EIGEN_INCLUDE_PATH=/usr/include/eigen3 \
-D WITH_OPENCL=OFF \
-D WITH_CUDA=ON \
-D CUDA_ARCH_BIN=5.3 \
-D CUDA_ARCH_PTX="" \
-D WITH_CUDNN=ON \
-D WITH_CUBLAS=ON \
-D ENABLE_FAST_MATH=ON \
-D CUDA_FAST_MATH=ON \
-D OPENCV_DNN_CUDA=ON \
-D ENABLE_NEON=ON \
-D WITH_QT=OFF \
-D WITH_OPENMP=ON \
-D WITH_OPENGL=ON \
-D BUILD_TIFF=ON \
-D WITH_FFMPEG=ON \
-D WITH_GSTREAMER=ON \
-D WITH_TBB=ON \
-D BUILD_TBB=ON \
-D BUILD_TESTS=OFF \
-D WITH_EIGEN=ON \
-D WITH_V4L=ON \
-D WITH_LIBV4L=ON \
-D OPENCV_ENABLE_NONFREE=ON \
-D INSTALL_C_EXAMPLES=OFF \
-D INSTALL_PYTHON_EXAMPLES=OFF \
-D BUILD_NEW_PYTHON_SUPPORT=ON \
-D BUILD_opencv_python3=TRUE \
-D OPENCV_GENERATE_PKGCONFIG=ON \
-D BUILD_EXAMPLES=OFF ..
I got this message when everything was done building.
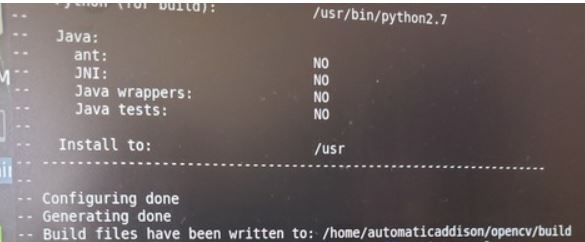
Build OpenCV. This command below will take a long time (1-2 hours), so you can go do something else and come back later.
make -j4
If the building process stops before it reaches 100%, repeat the cmake command I showed earlier, and run the ‘make -j4’ command again.
One other thing. A lot of times I had the installation stall. To avoid that happening, I moved the mouse cursor every few minutes so that the screen saver for the Jetson Nano didn’t turn on.
Finish the install.
cd ~
sudo rm -r /usr/include/opencv4/opencv2
cd ~/opencv/build
sudo make install
sudo ldconfig
make clean
sudo apt-get update
Verify Your OpenCV Installation
Let’s verify that everything is working correctly.
python3
import cv2
cv2.__version__
exit()
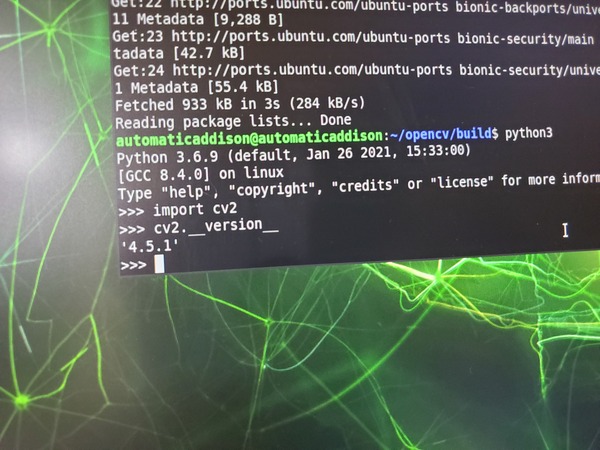
Final Housekeeping
Delete the original OpenCV and OpenCV_Contrib folders.
sudo rm -rf ~/opencv
sudo rm -rf ~/opencv_contrib
Install jtop, a system monitoring software for Jetson Nano.
cd ~
sudo -H pip3 install -U jetson-stats
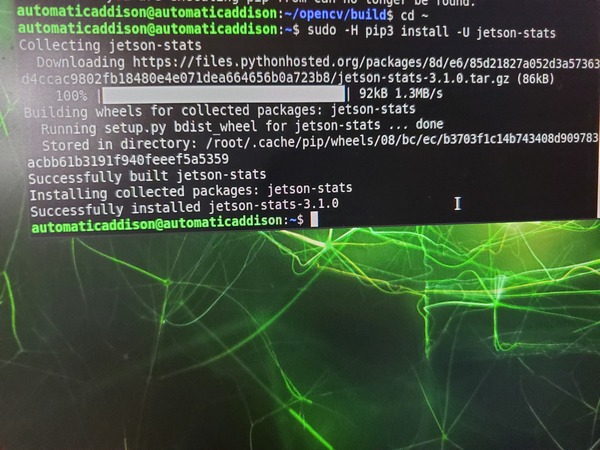
Reboot your machine.
sudo reboot
jtop
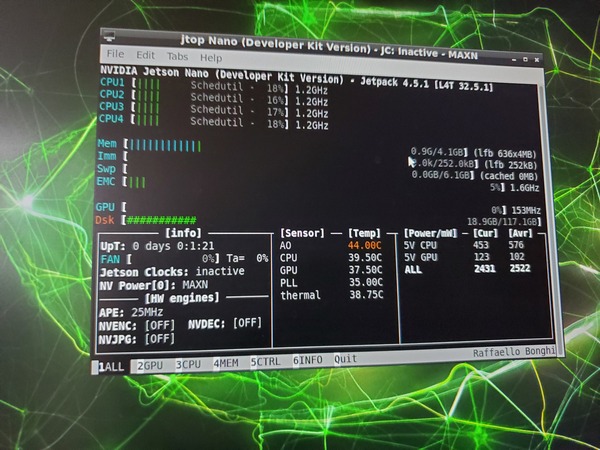
Press q to quit.
Verify the installation of OpenCV one last time.
python3
import cv2
cv2.__version__
exit()
That’s it. Keep building!
References
This article over at Q-engineering was really helpful.