Learning and true intelligence are more than classification and regression (which is what deep learning is really good at). Deep learning is deficient in perhaps one of the most important types of intelligence, emotional intelligence. Deep learning cannot negotiate trade agreements between countries, resolve conflict between a couple going through a divorce, or craft legislation to reduce student debt.
The long-run viability of deep learning as well as progress towards machines that are more intelligent will depend both on improvements in computing power as well as our ability to understand and subsequently quantify general intelligence.
Concerning the quantification of general intelligence, this really is the inherent goal of not just artificial intelligence, but of computer science as a whole. We want a machine that can automate tasks that humans do and to be as independent as possible while doing it. We want to teach a computer to think the way a human thinks, to understand the way a human understands, and to infer the way a human infers. At present, the state-of-the-art is a long way from achieving this goal.
Deep learning is only as good as the data fed to it: garbage in, garbage out. Deep learning has a lot of limitations and is a long way from the kind of artificial general intelligence envisioned in those science fiction movies.
At this point, the state of the art in deep learning is identifying images, detecting fraud, processing audio, and performing advertising. The only thing that we really know for sure about deep learning at this point is that it performs some tasks at a very high level, and we aren’t 100% sure why it does. We understand the probability and the algorithm behind the representations that we are making, but we don’t understand what the network is actually learning like we would understand a classification tree or a run of Naive Bayes.
Understanding what abstractions that the network is learning is essential to move forward in the field. If at some point the theory is proven to be invalid, then all the emerging techniques will be rendered useless.
In any case, even if some theoretical basis is proven, it may or may not show a strong relationship that warrants continued development in these fields.
Deep Learning Needs Generalists Not Just Specialists
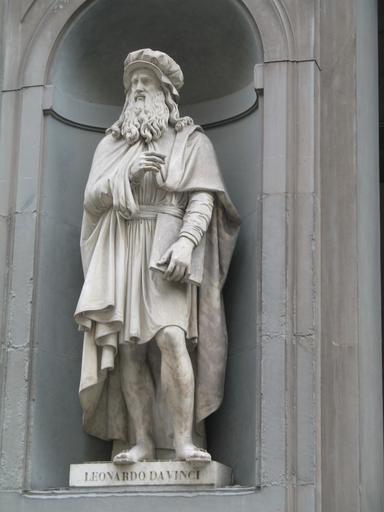
My prediction is that the real breakthroughs on the route to artificial general intelligence will come from the T-shaped generalists, those professionals who have the breadth and depth to see the unseen interconnectedness.
In order for deep learning and AI to be viable over the long run, researchers in this field will need to be able to think outside the box and be well-versed in the fundamentals of multiple disciplines, not just their own silo. Mathematicians need to learn a little bit about computer science, neuroscience, and psychology. Computer scientists need to learn a little bit about neuroscience and psychology. Neuroscientists need to cross over and learn a little bit about computer science as well as mathematics.
While learning about other fields is no guarantee that a researcher will make a breakthrough, staying in a narrow area and not venturing out is a guarantee of not making a breakthrough. As they say, to the hammer, everything looks like a nail.
As a final note, I’m keeping my eye out on quantum computing, and, more importantly, quantum machine learning and quantum neural networks. Quantum machine learning entails executing machine learning algorithms on a quantum computer. It will be interesting to see how getting away from the 1s and 0s of a standard computer will impact the field, and if it will usher in a new era of artificial intelligence. Only time will tell.