In this tutorial, we’ll learn the basics of how to interface ROS 2 with OpenCV, the popular computer vision library. These basics will provide you with the foundation to add vision to your robotics applications.
We’ll create an image publisher node to publish webcam data (i.e. video frames) to a topic, and we’ll create an image subscriber node that subscribes to that topic.
Prerequisites
- ROS 2 Foxy Fitzroy installed on Ubuntu Linux 20.04
- You have already created a ROS 2 workspace.
- You have a working webcam that is connected and tested on your Ubuntu installation.
- You are able to run the code on this tutorial on your Ubuntu machine without any problems.
Create a Package
Open a new terminal window, and navigate to the src directory of your workspace:
cd ~/dev_ws/src
Now let’s create a package named cv_basics.
Type this command (this is all a single command):
ros2 pkg create --build-type ament_python cv_basics --dependencies rclpy image_transport cv_bridge sensor_msgs std_msgs opencv2
Modify Package.xml
Go to the dev_ws/src/cv_basics folder.
cd ~/dev_ws/src/cv_basics
Make sure you have a text editor installed. I like to use gedit.
sudo apt-get install gedit
Open the package.xml file.
gedit package.xml
Fill in the description of the cv_basics package, your email address and name on the maintainer line, and the license you desire (e.g. Apache License 2.0).
<description>A minimal image publisher and subscriber node that uses OpenCV</description>
<maintainer email="automaticaddison@todo.todo">automaticaddison</maintainer>
<license>Apache License 2.0</license>
Save and close the file.
Create the Image Publisher Node (Python)
Move to the cv_basics package.
cd ~/dev_ws/src/cv_basics/cv_basics
Open a new Python file named webcam_pub.py.
gedit webcam_pub.py
Type the code below into it:
# Basic ROS 2 program to publish real-time streaming
# video from your built-in webcam
# Author:
# - Addison Sears-Collins
# - https://automaticaddison.com
# Import the necessary libraries
import rclpy # Python Client Library for ROS 2
from rclpy.node import Node # Handles the creation of nodes
from sensor_msgs.msg import Image # Image is the message type
from cv_bridge import CvBridge # Package to convert between ROS and OpenCV Images
import cv2 # OpenCV library
class ImagePublisher(Node):
"""
Create an ImagePublisher class, which is a subclass of the Node class.
"""
def __init__(self):
"""
Class constructor to set up the node
"""
# Initiate the Node class's constructor and give it a name
super().__init__('image_publisher')
# Create the publisher. This publisher will publish an Image
# to the video_frames topic. The queue size is 10 messages.
self.publisher_ = self.create_publisher(Image, 'video_frames', 10)
# We will publish a message every 0.1 seconds
timer_period = 0.1 # seconds
# Create the timer
self.timer = self.create_timer(timer_period, self.timer_callback)
# Create a VideoCapture object
# The argument '0' gets the default webcam.
self.cap = cv2.VideoCapture(0)
# Used to convert between ROS and OpenCV images
self.br = CvBridge()
def timer_callback(self):
"""
Callback function.
This function gets called every 0.1 seconds.
"""
# Capture frame-by-frame
# This method returns True/False as well
# as the video frame.
ret, frame = self.cap.read()
if ret == True:
# Publish the image.
# The 'cv2_to_imgmsg' method converts an OpenCV
# image to a ROS 2 image message
self.publisher_.publish(self.br.cv2_to_imgmsg(frame))
# Display the message on the console
self.get_logger().info('Publishing video frame')
def main(args=None):
# Initialize the rclpy library
rclpy.init(args=args)
# Create the node
image_publisher = ImagePublisher()
# Spin the node so the callback function is called.
rclpy.spin(image_publisher)
# Destroy the node explicitly
# (optional - otherwise it will be done automatically
# when the garbage collector destroys the node object)
image_publisher.destroy_node()
# Shutdown the ROS client library for Python
rclpy.shutdown()
if __name__ == '__main__':
main()
Save and close the editor.
Modify Setup.py
Go to the following directory.
cd ~/dev_ws/src/cv_basics/
Open setup.py.
gedit setup.py
Add the following line between the ‘console_scripts’: brackets:
'img_publisher = cv_basics.webcam_pub:main',
entry_points={
'console_scripts': [
'img_publisher = cv_basics.webcam_pub:main',
],
},
Save the file, and close it.
Create the Image Subscriber Node (Python)
Move to the dev_ws/src/cv_basics/cv_basics folder.
cd ~/dev_ws/src/cv_basics/cv_basics
Now, let’s create the subscriber node. We’ll name it webcam_sub.py.
gedit webcam_sub.py
Type the code below into it:
# Basic ROS 2 program to subscribe to real-time streaming
# video from your built-in webcam
# Author:
# - Addison Sears-Collins
# - https://automaticaddison.com
# Import the necessary libraries
import rclpy # Python library for ROS 2
from rclpy.node import Node # Handles the creation of nodes
from sensor_msgs.msg import Image # Image is the message type
from cv_bridge import CvBridge # Package to convert between ROS and OpenCV Images
import cv2 # OpenCV library
class ImageSubscriber(Node):
"""
Create an ImageSubscriber class, which is a subclass of the Node class.
"""
def __init__(self):
"""
Class constructor to set up the node
"""
# Initiate the Node class's constructor and give it a name
super().__init__('image_subscriber')
# Create the subscriber. This subscriber will receive an Image
# from the video_frames topic. The queue size is 10 messages.
self.subscription = self.create_subscription(
Image,
'video_frames',
self.listener_callback,
10)
self.subscription # prevent unused variable warning
# Used to convert between ROS and OpenCV images
self.br = CvBridge()
def listener_callback(self, data):
"""
Callback function.
"""
# Display the message on the console
self.get_logger().info('Receiving video frame')
# Convert ROS Image message to OpenCV image
current_frame = self.br.imgmsg_to_cv2(data)
# Display image
cv2.imshow("camera", current_frame)
cv2.waitKey(1)
def main(args=None):
# Initialize the rclpy library
rclpy.init(args=args)
# Create the node
image_subscriber = ImageSubscriber()
# Spin the node so the callback function is called.
rclpy.spin(image_subscriber)
# Destroy the node explicitly
# (optional - otherwise it will be done automatically
# when the garbage collector destroys the node object)
image_subscriber.destroy_node()
# Shutdown the ROS client library for Python
rclpy.shutdown()
if __name__ == '__main__':
main()
Save and close the editor.
Modify Setup.py
Go to the following directory.
cd ~/dev_ws/src/cv_basics/
Open setup.py.
gedit setup.py
Make sure the entry_points block looks like this:
entry_points={
'console_scripts': [
'img_publisher = cv_basics.webcam_pub:main',
'img_subscriber = cv_basics.webcam_sub:main',
],
},
Save the file, and close it.
Build the Package
Return to the root of your workspace:
cd ~/dev_ws/
We need to double check that all the dependencies needed are already installed.
rosdep install -i --from-path src --rosdistro foxy -y
If you get the following error:
ERROR: the following packages/stacks could not have their rosdep keys resolved to system dependencies: cv_basics: Cannot locate rosdep definition for [opencv2]
Follow these instructions to resolve the error.
Build the package:
colcon build --packages-select cv_basics
Run the Nodes
To run the nodes, open a new terminal window.
Make sure you are in the root of your workspace:
cd ~/dev_ws/
Run the publisher node. If you recall, its name is img_publisher.
ros2 run cv_basics img_publisher
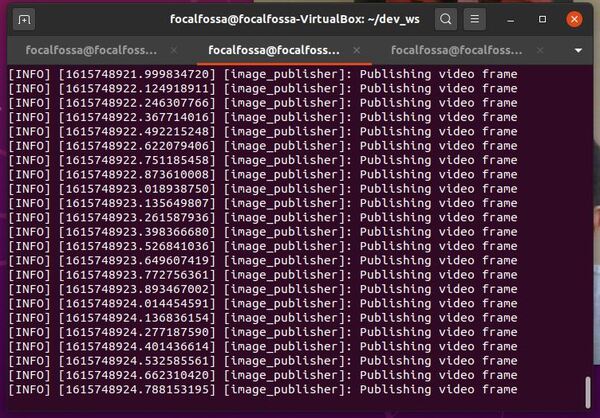
A window will pop up with the streaming video.
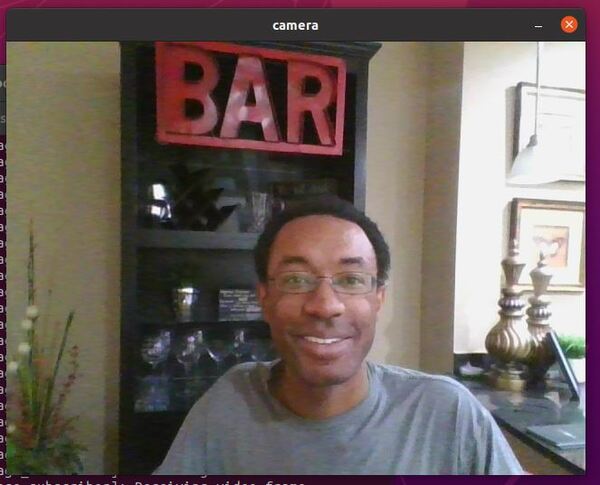
Open a new terminal, and run the subscriber node.
ros2 run cv_basics img_subscriber
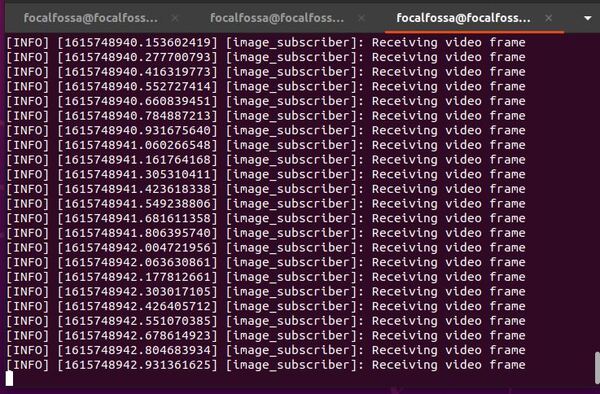
Check out the active topics.
ros2 topic list -t

See the relationship between the active nodes.
rqt_graph
Click the refresh button. The refresh button is that circular arrow at the top of the screen.

We can see that the image_publisher node is publishing images to the video_frames topic. The image_subscriber node is subscribing to those video frames that are published to the video_frames topic.
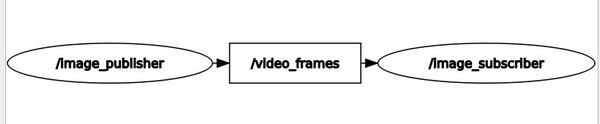
That’s it! Keep building!