In this tutorial, we will focus on lists in Python, a versatile data structure that is especially useful in robotics for handling collections of items like sensor readings or robotic commands.
Prerequisites
- You have completed this tutorial: How to Make Decisions in Code Using Python.
Working with Lists
Let’s create a new file named robotics_lists.py inside the following folder: ~/Documents/python_tutorial.
cd ~/Documents/python_tutorial
code .
First, let’s define a list to store sensor data.
# Sensor data list
sensor_readings = [20, 55, 75, 10]
print("Initial Sensor Readings:", sensor_readings)
This list holds some dummy sensor readings.
To access an element, use its index. Here’s how you access the first and the last reading:
# Accessing elements
print("First reading:", sensor_readings[0]) # 20
print("Most recent reading:", sensor_readings[-1]) # 10
Suppose a sensor is recalibrated, and you need to update its reading:
# Updating a sensor reading
sensor_readings[2] = 80
print("Updated Readings:", sensor_readings)
Adding new sensor data as it comes can be achieved with append:
# Adding a new sensor reading
sensor_readings.append(65)
print("Readings after append:", sensor_readings)
If a sensor malfunctions, you might need to remove its data:
# Removing a sensor reading
del sensor_readings[0] # Removing the first reading
print("Readings after deletion:", sensor_readings)
To handle each reading, say for analysis or adjustment, iterate through the list:
# Iterating through sensor readings
for reading in sensor_readings:
print("Processing reading:", reading)
Now run the code.
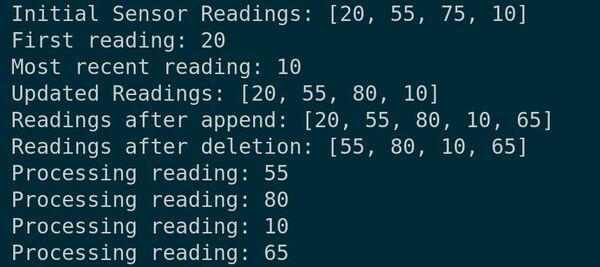
Creating a Set
Now let’s explore sets in Python. Sets are perfect for managing unique items in robotics, like tracking visited locations or unique sensor IDs.”
Create a new file named robotics_sets.py inside the following folder: ~/Documents/python_tutorial.
Type the following code into the editor.
# Creating a set of unique sensor IDs
sensor_ids = {101, 102, 103, 104}
print("Unique Sensor IDs:", sensor_ids)
Here we define a set with unique sensor IDs. Sets are great because they automatically handle uniqueness.
Now, let’s see what happens when we try to add duplicates.
# Attempting to add duplicate sensor IDs
sensor_ids.update([102, 103, 105])
print("Updated Sensor IDs:", sensor_ids)
Notice how it ignores duplicates but adds new unique items.
To add new sensor data or remove outdated ones, we use add() and discard().
# Managing sensor data
sensor_ids.add(106)
print("After adding:", sensor_ids)
sensor_ids.discard(101)
print("After discarding:", sensor_ids)
discard() is safe to use because it won’t cause an error if the item doesn’t exist, unlike remove().
We can also perform set operations like unions and intersections, useful for comparing sensor data across different sets.
# Set operations with sensor data
another_set = {104, 105, 107}
print("Union:", sensor_ids.union(another_set))
print("Intersection:", sensor_ids.intersection(another_set))
These operations help determine overlaps or combining data from multiple sensor sets.
Run the script.
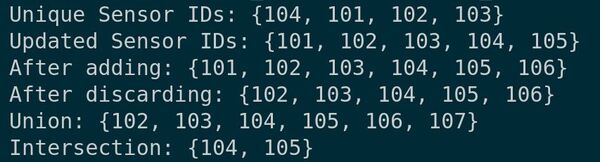
You’ll observe how sets efficiently manage unique items and perform operations that are important in robotics for data handling.
Creating a Tuple
Let’s dive into tuples in Python. Tuples are immutable collections, meaning once created, you can’t change their elements, making them ideal for data that should remain constant.
Let’s create a new file named using_tuples.py inside the following folder: ~/Documents/python_tutorial.
Tuples are defined by enclosing elements in parentheses. Let’s create one now.
# Creating a tuple
coordinates = (10, 20, 30)
print("Coordinates:", coordinates)
This tuple could represent coordinates in 3D space, for example.
Tuples are great for accessing elements by index:
# Accessing tuple elements
print("X-coordinate:", coordinates[0])
print("Z-coordinate:", coordinates[-1])
Tuples can also hold mixed data types and can be unpacked into separate variables, which is very handy in many situations.
# Tuple with mixed data types and unpacking
info_tuple = ("Item", 150, 23.99)
name, quantity, price = info_tuple
print("Unpacked:", name, quantity, price)
Save your file, and let’s run it to demonstrate these tuple features.
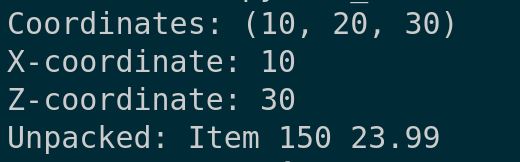
You can see how we use tuples to store and handle data securely and efficiently.
Creating a Dictionary
Let’s explore how to create and use dictionaries in Python. Dictionaries are a fundamental data structure for storing key-value pairs, which is useful for managing complex information.
Let’s start by creating a new file named creating_a_dictionary.py inside the following folder: ~/Documents/python_tutorial.
Here’s how to define a dictionary with curly braces, using keys and values.
Type the following code into the editor:
# Creating a dictionary
robot_parts = {'wheels': 4, 'motors': 2, 'sensors': 5}
print("Robot Parts Dictionary:", robot_parts)
print("")
This example creates a dictionary to track robot components.
Now, let’s make a more complex dictionary, including lists and other dictionaries as values.
# Dictionary with various data types
robot_specs = {
'name': 'AutomaticAddison Robot',
'parts': robot_parts,
'features': ['autonomous', 'solar-powered', 'waterproof'],
'dimensions': {'height': 120, 'width': 75, 'weight': 150}
}
print("Robot Specifications Dictionary:", robot_specs)
print("")
To access or modify dictionary data, reference the keys.
# Accessing and modifying dictionary values
print("Robot Height:", robot_specs['dimensions']['height'])
print("")
robot_specs['speed'] = '25 km/h'
print("Updated Robot Specifications:", robot_specs)
print("")
# Removing an item
del robot_specs['speed']
print("After deletion:", robot_specs)
print("")
Finally, dictionaries can be traversed using loops to access all keys, values, or both.
# Looping through a dictionary
for key, value in robot_specs.items():
print(key, ":", value)
Now, let’s run the script to see how dictionaries function.
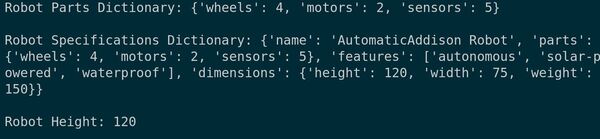
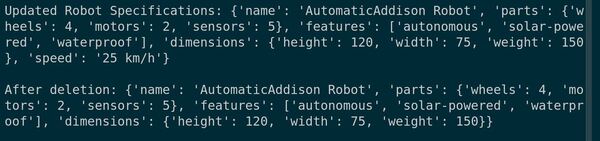

You’ll observe how effectively dictionaries store and manage complex data.
That’s a quick guide on creating and using dictionaries in Python.
Thanks for following along with me, and I’ll see you in the next tutorial.
Keep building!