In this tutorial, we’ll explore how to create pick and place applications using the MoveIt Task Constructor for ROS 2. The MoveIt Task Constructor allows us to define complex robotic tasks as a series of modular stages, making our motion planning more flexible and easier to maintain.
We’ll build two applications from scratch that demonstrate how to pick up an object from one location and place it in another, showcasing the power and versatility of the Task Constructor.
Here is what you will be able to create by the end of this tutorial:
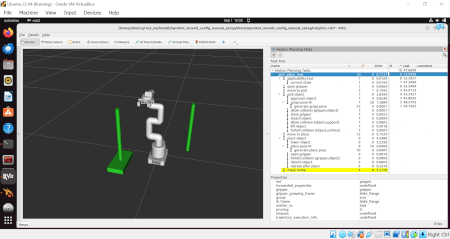
And you will have a digital twin that enable you to execute the same motions in Gazebo to execute pick and place in a simulated world:
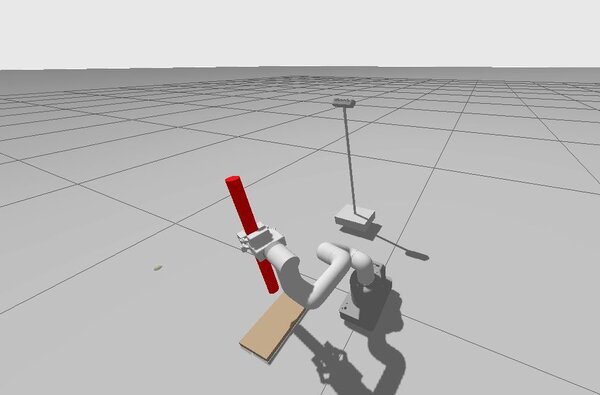
Our pick and place applications will demonstrate:
- Setting up a pick and place task with multiple stages
- Using both Cartesian and sampling-based motion planners
- Integrating with ROS 2 and providing detailed feedback on the planning process
- Handling collisions and modifying the planning scene
Here’s a high-level overview of what our programs will do:
- Set up the demo scene with a table and an object to be picked
- Define a pick sequence that includes:
- Opening the gripper
- Moving to a pre-grasp position
- Approaching the object
- Closing the gripper
- Lifting the object
- Define a place sequence that includes:
- Moving to the place location
- Lowering the object
- Opening the gripper
- Retreating from the placed object
- Plan the entire pick and place task
- Optionally execute the planned task
- Provide detailed feedback on each stage of the process
This tutorial will give you a solid foundation and template for using the MoveIt Task Constructor for complex manipulation tasks, which you can then adapt and expand for your specific pick and place applications.
Real-World Use Cases
The pick and place applications you’ll develop in this tutorial using the MoveIt Task Constructor have numerous practical applications across various industries:
- Manufacturing and Assembly
- Automate the handling of components on production lines
- Pick parts from conveyors or bins and place them precisely for assembly
- Adapt to different product variants by modifying pick and place parameters
- Warehouse and Logistics
- Sort and organize packages of various sizes and weights
- Load and unload containers or pallets with diverse items
- Integrate with vision systems for flexible item recognition and grasping
- Food and Beverage Industry
- Handle delicate items like fruits or baked goods without damage
- Pick and place items for packaging or quality control inspection
- E-commerce and Retail
- Pick items from inventory for order fulfillment
- Sort returned items for restocking or processing
- Pack items into boxes or bags for shipping
Prerequisites
- You have completed this tutorial.
All the code is here on my GitHub repository. Note that I am working with ROS 2 Iron, so the steps might be slightly different for other versions of ROS 2.
Example 1
Create Include File
Open a new terminal window, and type:
cd ~/ros2_ws/src/mycobot_ros2/hello_moveit_task_constructor/include/hello_moveit_task_constructor
gedit pick_place_task.h
Add this code.
/**
* @file pick_place_task.hpp
* @brief Defines the PickPlaceTask class for a pick and place demo using MoveIt Task Constructor.
*
* This file contains the declaration of the PickPlaceTask class, which sets up and executes
* a pick and place task using MoveIt Task Constructor (MTC). It also includes a function
* to set up the demo scene.
*
* @author Addison Sears-Collins
* @date August 21, 2024
*/
#pragma once
// Include necessary ROS 2 headers
#include <rclcpp/node.hpp>
#include <rclcpp/rclcpp.hpp>
// Include MoveIt headers
#include <moveit/planning_scene/planning_scene.h>
#include <moveit/robot_model/robot_model.h>
#include <moveit/planning_scene_interface/planning_scene_interface.h>
// Include MoveIt Task Constructor (MTC) headers
#include <moveit/task_constructor/container.h>
#include <moveit/task_constructor/properties.h>
#include <moveit/task_constructor/task.h>
#include <moveit/task_constructor/stages/compute_ik.h>
#include <moveit/task_constructor/stages/connect.h>
#include <moveit/task_constructor/stages/current_state.h>
#include <moveit/task_constructor/stages/generate_grasp_pose.h>
#include <moveit/task_constructor/stages/generate_pose.h>
#include <moveit/task_constructor/stages/generate_place_pose.h>
#include <moveit/task_constructor/stages/modify_planning_scene.h>
#include <moveit/task_constructor/stages/move_relative.h>
#include <moveit/task_constructor/stages/move_to.h>
#include <moveit/task_constructor/stages/predicate_filter.h>
#include <moveit/task_constructor/solvers/cartesian_path.h>
#include <moveit/task_constructor/solvers/joint_interpolation.h>
#include <moveit/task_constructor/solvers/pipeline_planner.h>
#include <moveit_task_constructor_msgs/action/execute_task_solution.hpp>
// Include the generated parameters header
// (generated from src/pick_place_demo_parameters.yaml)
#include "pick_place_demo_parameters.hpp"
namespace hello_moveit_task_constructor {
// Using directive for convenience
using namespace moveit::task_constructor;
/**
* @brief Set up the demo scene using ROS parameters.
*
* This function prepares the demo environment based on the provided parameters.
*
* @param params The parameters for the pick and place demo.
*/
void setupDemoScene(const pick_place_demo::Params& params);
/**
* @brief Print detailed information about a stage and its substages.
*
* This function recursively prints information about a given stage and all of its substages.
* It includes details such as the number of solutions, failures, and specific failure messages.
* The output is indented to reflect the hierarchical structure of the stages.
*
* @param stage Pointer to the Stage object to be printed.
* @param indent The indentation level for the current stage (default is 0).
*/
void printStageDetails(const moveit::task_constructor::Stage* stage, int indent = 0);
/**
* @class PickPlaceTask
* @brief Represents a pick and place task using MoveIt Task Constructor.
*
* This class encapsulates the functionality to set up, plan, and execute
* a pick and place task using MoveIt Task Constructor.
*/
class PickPlaceTask
{
public:
/**
* @brief Construct a new PickPlaceTask object.
*
* @param task_name The name of the task.
*/
PickPlaceTask(const std::string& task_name);
/**
* @brief Destroy the PickPlaceTask object.
*/
~PickPlaceTask() = default;
/**
* @brief Initialize the pick and place task.
*
* @param node The ROS 2 node.
* @param params The parameters for the pick and place demo.
* @return true if initialization was successful, false otherwise.
*/
bool init(const rclcpp::Node::SharedPtr& node, const pick_place_demo::Params& params);
/**
* @brief Plan the pick and place task.
*
* @param max_solutions The maximum number of solutions to generate.
* @return true if planning was successful, false otherwise.
*/
bool plan(const std::size_t max_solutions);
/**
* @brief Execute the planned pick and place task.
*
* @return true if execution was successful, false otherwise.
*/
bool execute();
private:
// The name of the task
std::string task_name_;
// Pointer to the MoveIt Task Constructor task
moveit::task_constructor::TaskPtr task_;
};
} // namespace hello_moveit_task_constructor
Save the file, and close it.
pick_place_task.h is the header file that declares the PickPlaceTask class. It defines the interface for initializing, planning, and executing a pick and place operation using the MoveIt Task Constructor. This header includes method declarations for setting up the task pipeline, planning the motion, and executing the planned trajectory.
Create the Code
Open a new terminal window, and type:
cd ~/ros2_ws/src/mycobot_ros2/hello_moveit_task_constructor/src/
gedit pick_place_demo.cpp
Add this code.
/**
* @file pick_place_demo.cpp
* @brief Main entry point for the pick and place demo using MoveIt Task Constructor.
*
* This program sets up and runs a pick and place task demonstration using the
* MoveIt Task Constructor framework. It initializes the ROS 2 node, sets up the
* demo scene, plans the pick and place task, and optionally executes it.
*
* @author Addison Sears-Collins
* @date August 21, 2024
*/
#include <rclcpp/rclcpp.hpp>
// Include the pick/place task implementation
#include <hello_moveit_task_constructor/pick_place_task.h>
#include "pick_place_demo_parameters.hpp" // Automatically generated from the yaml file pick_place_demo_parameters.yaml
// Set up a logger for this demo
static const rclcpp::Logger LOGGER = rclcpp::get_logger("pick_place_demo");
int main(int argc, char** argv) {
// Initialize ROS 2
rclcpp::init(argc, argv);
// Set up node options to automatically declare parameters
rclcpp::NodeOptions node_options;
node_options.automatically_declare_parameters_from_overrides(true);
// Create a ROS 2 node for this demo
auto node = rclcpp::Node::make_shared("pick_place_demo", node_options);
// Start a separate thread to handle ROS 2 callbacks
std::thread spinning_thread([node] { rclcpp::spin(node); });
// Create a parameter listener to get the demo parameters
const auto param_listener = std::make_shared<pick_place_demo::ParamListener>(node);
const auto params = param_listener->get_params();
// Set up the demo scene based on the parameters
hello_moveit_task_constructor::setupDemoScene(params);
// Create the pick and place task
hello_moveit_task_constructor::PickPlaceTask pick_place_task("pick_place_task");
// Initialize the pick and place task
if (!pick_place_task.init(node, params)) {
RCLCPP_INFO(LOGGER, "Initialization failed");
return 1;
}
// Plan the pick and place task
if (pick_place_task.plan(params.max_solutions)) {
RCLCPP_INFO(LOGGER, "Planning succeeded");
// Execute the plan if execution is enabled in the parameters
if (params.execute) {
pick_place_task.execute();
RCLCPP_INFO(LOGGER, "Execution complete");
} else {
RCLCPP_INFO(LOGGER, "Execution disabled");
}
} else {
RCLCPP_INFO(LOGGER, "Planning failed");
}
// Wait for the spinning thread to finish (keeps the node alive for introspection)
spinning_thread.join();
return 0;
}
Save the file, and close it.
pick_place_demo.cpp serves as the main entry point for the demo. It initializes the ROS node, loads parameters from the YAML file, sets up the demo scene, and orchestrates the execution of the pick and place task. This file bridges the configuration data with the task implementation, demonstrating how to use the PickPlaceTask class in a ROS environment.
Open a new terminal window, and type:
cd ~/ros2_ws/src/mycobot_ros2/hello_moveit_task_constructor/src/
gedit pick_place_task.cpp
Add this code.
Save the file, and close it.
pick_place_task.cpp implements the PickPlaceTask class defined in the header. It constructs the task pipeline using MoveIt Task Constructor stages such as current state, move relative, generate grasp pose, compute IK (inverse kinematics), and modify the planning scene. This file translates the high-level pick and place task into a sequence of robot motions and actions.
Create a Parameter File
Open a new terminal window, and type:
cd ~/ros2_ws/src/mycobot_ros2/hello_moveit_task_constructor/src/
gedit pick_place_demo_parameters.yaml
Add this code.
pick_place_demo:
execute:
type: bool
default_value: false
controller_names:
type: string_array
default_value: ["arm_controller", "grip_action_controller"]
validation:
not_empty<>: []
table_name:
type: string
default_value: "table"
validation:
not_empty<>: []
table_reference_frame:
type: string
default_value: "base_link"
validation:
not_empty<>: []
table_dimensions:
type: double_array
default_value: [0.10, 0.20, 0.03]
validation:
fixed_size<>: [3]
table_pose:
type: double_array
default_value: [0.22, 0.12, 0.0, 0.0, 0.0, 0.0]
validation:
fixed_size<>: [6]
object_name:
type: string
default_value: "object"
validation:
not_empty<>: []
object_reference_frame:
type: string
default_value: "base_link"
validation:
not_empty<>: []
object_dimensions:
type: double_array
default_value: [0.35, 0.0125] # [height, radius] of cylinder
validation:
fixed_size<>: [2]
object_pose:
type: double_array
default_value: [0.22, 0.12, 0.0, 0.0, 0.0, 0.0]
validation:
fixed_size<>: [6]
spawn_table:
type: bool
default_value: true
max_solutions:
type: int
default_value: 10
arm_group_name:
type: string
default_value: "arm"
validation:
not_empty<>: []
eef_name:
type: string
default_value: "gripper"
validation:
not_empty<>: []
gripper_group_name:
type: string
default_value: "gripper"
validation:
not_empty<>: []
gripper_frame:
type: string
default_value: "link6_flange"
validation:
not_empty<>: []
gripper_open_pose:
type: string
default_value: "open"
validation:
not_empty<>: []
gripper_close_pose:
type: string
default_value: "half_closed"
validation:
not_empty<>: []
arm_home_pose:
type: string
default_value: "home"
validation:
not_empty<>: []
# Scene frames
world_frame:
type: string
default_value: "base_link"
validation:
not_empty<>: []
surface_link:
type: string
default_value: "table"
validation:
not_empty<>: []
grasp_frame_transform:
type: double_array
default_value: [0.0, 0.0, 0.096, 1.5708, 0.0, 0.0]
validation:
fixed_size<>: [6]
place_pose:
type: double_array
default_value: [-0.183, -0.14, 0.0, 0.0, 0.0, 0.0]
validation:
fixed_size<>: [6]
place_surface_offset:
type: double
default_value: -0.03 # -0.03 Enables the cylinder to stand on the floor
approach_object_min_dist:
type: double
default_value: 0.0015
approach_object_max_dist:
type: double
default_value: 0.3
lift_object_min_dist:
type: double
default_value: 0.005
lift_object_max_dist:
type: double
default_value: 0.3
Save the file, and close it.
pick_place_demo_parameters.yaml is a configuration file that defines all parameters for the pick and place operation. It includes robot specifications, object properties, scene setup, and motion planning parameters. This YAML file allows for modification of the demo setup without altering the core code.
Build the Code
cd ~/ros2_ws/
colcon build
source ~/.bashrc
(OR source ~/ros2_ws/install/setup.bash if you haven’t set up your bashrc file to source your ROS distribution automatically with “source ~/ros2_ws/install/setup.bash”)
Launch
Open two terminal windows, and run the following commands to launch our standard MoveIt 2 environment:
ros2 launch mycobot_gazebo mycobot_280_arduino_bringup_ros2_control_gazebo.launch.py use_rviz:=false
ros2 launch hello_moveit_task_constructor demo.launch.py
Now run the demo:
ros2 launch hello_moveit_task_constructor run.launch.py exe:=pick_place_demo
Here is what you should see:
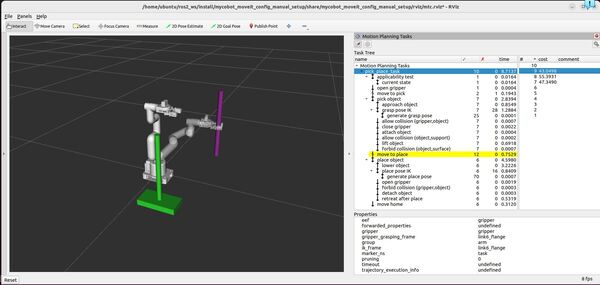
Understanding the Motion Planning Results
RViz – “Motion Planning Tasks” Panel
The “Motion Planning Tasks” panel in RViz provides a detailed breakdown of our pick and place task. It presents a hierarchical view with “Motion Planning Tasks” at the root, followed by “pick_place_task”.
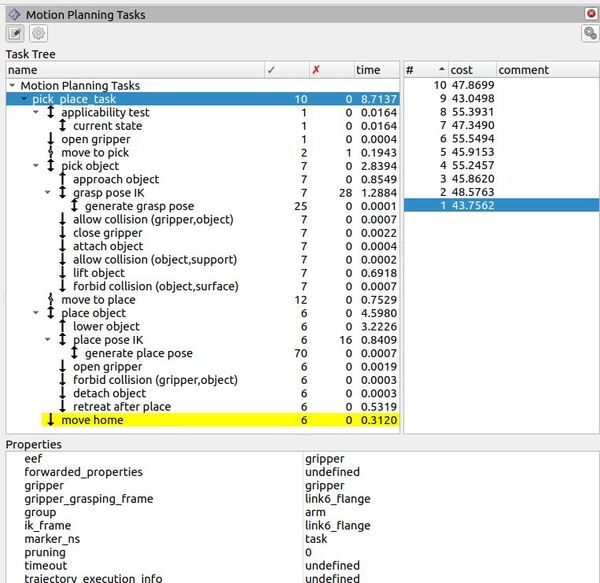
Under “pick_place_task”, we can see the following stages:
- “applicability test“: This initial stage checks if the task can be executed in the current state.
- “current state“: Represents the initial state of the robot.
- “open gripper“: The first movement to open the gripper before picking.
- “move to pick“: Moving the arm to the pre-grasp position.
- “pick object“: A container for all pick-related stages, including:
- “approach object”: Moving towards the object
- “grasp pose IK”: Calculating inverse kinematics for the grasp
- “allow collision (gripper,object)”: Temporarily allowing collision between the gripper and object
- “close gripper”: Closing the gripper to grasp the object
- “attach object”: Attaching the object to the robot model
- “allow collision (object,support)”: Allowing collision between the object and its support surface
- “lift object”: Lifting the grasped object
- “forbid collision (object,surface)”: Preventing collision between the object and surface after lifting
- “move to place“: Moving the arm to the pre-place position.
- “place object“: A container for all place-related stages, similar in structure to “pick object”.
- “move home“: The final movement to return the robot to its home position.
The second column shows green checkmarks for each stage, indicating that every step of the plan was successfully computed. The numbers (ranging from 1 to 28) indicate how many solutions were found for each stage.
The “time” column displays the computational time for each component. We can see that the entire “pick_place_task” took 8.7137 seconds to compute, with individual stages taking varying amounts of time.
The “cost” column represents a metric used by the motion planner to evaluate the quality of the solution. Lower costs generally indicate more efficient movements.
The “#” column shows the number of solutions propagated to the next stage, providing insight into the planner’s decision-making process.
The yellow highlighting on the “move home” stage indicates that this is the currently selected or focused stage in the RViz interface.
This breakdown allows us to verify that our pick and place task is structured correctly, with appropriate stages for picking, moving, placing, and returning home. It also provides valuable information about the planning process, including computation times and solution quality for each stage.
Terminal Window – Planning Results
If you look at the terminal window, you’ll see the detailed planning results.
Let’s interpret these outputs.
The MoveIt Task Constructor uses a hierarchical planning approach. It breaks down the overall pick and place task into smaller, manageable stages and plans each stage individually while considering the connections between them.
- Stage Creation: The terminal output shows each stage being added to the task, including the creation of various planners (OMPL, Joint Interpolation, Cartesian) and the initialization of the task pipeline.
- Planning Process: After all stages are set up, the planning process begins. We can see multiple calls to PipelinePlanner::plan, indicating that the planner is working on different stages of the task.
Let’s analyze some key parts of the output:
- Task Solutions: The planner found 10 solutions for the entire pick_place_task. This indicates that the planner was able to find multiple valid ways to complete the task.
- Solution Costs: Each solution has an associated cost. Lower costs generally indicate more efficient solutions. The costs range from about 43 to 55 in this case.
- Stage Breakdown: The output provides a detailed breakdown of each stage in the pick and place task. For example, we can see results for stages like “applicability test”, “open gripper”, and “move to pick”. Some stages have multiple solutions, while others have only one.
- Detailed Stage Information: For complex stages like “pick object”, we see a breakdown of substages. This includes steps like “approach object”, “grasp pose IK”, and “lift object”. Each of these substages has its own set of solutions and potential failures.
- IK Challenges: The output reveals challenges in finding inverse kinematics (IK) solutions for certain poses. This is particularly evident in the “grasp pose IK” stage, where we see numerous failures before valid solutions are found.
- Collision Checking: There are instances where the planner detects and handles potential collisions. This is crucial for ensuring the safety of the robot and its environment.
- Cartesian Path Planning: We can see the results of Cartesian path planning for movements like approaching the object and retreating after placing it.
- Final Stages: The plan includes stages for releasing the object, such as “open gripper”, “detach object”, and “retreat after place”.
This detailed output allows us to understand the complexity of the pick and place task and how the MoveIt Task Constructor breaks it down into manageable pieces. It showcases the planner’s ability to handle various constraints, find multiple solutions, and deal with challenges like IK solving and collision avoidance. The successful generation of multiple solutions indicates that the planner has effectively created a robust and flexible plan for the pick and place task.
Analysis of the Results
Let’s break down what we did and what we learned from this pick and place project.
Our Approach
We created a pick and place task consisting of several stages:
- Open gripper
- Move to pick position
- Approach object
- Close gripper and grasp object
- Lift object
- Move to place position
- Lower object
- Open gripper and release object
- Retreat from placed object
- Move to home position
The Results: A Stage-by-Stage Breakdown
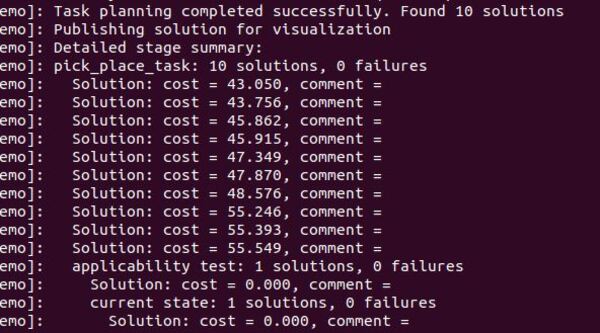
Looking at our terminal output and RViz Motion Planning Tasks panel, here’s what we observed:
Task Creation:
- Successfully added all stages of the pick and place task
- Created and initialized various planners (OMPL, Joint Interpolation, Cartesian)
Planning Process:
- The task planning completed successfully
- Found 10 solutions for the entire task
Detailed Task State:
- Root “pick_place_task”: 10 solutions, 0 failures
- Indicates multiple valid solutions were found for the entire task
- Individual Stages:
- “applicability test” and “current state”: 1 solution, 0 failures
- Successfully checked initial conditions
- “open gripper”: 1 solution, 0 failures
- Straightforward planning for gripper opening
- “move to pick”: 2 solutions, 1 failure
- Multiple solutions found, but also encountered a failure
- “pick object”: 7 solutions, 0 failures
- Complex stage with multiple substages, all successful
- “move to place”: 12 solutions, 0 failures
- Many valid paths found for this movement
- “place object”: 6 solutions, 0 failures
- Successfully planned object placement
- “move home”: 6 solutions, 0 failures
- Multiple paths found for returning to home position
- “applicability test” and “current state”: 1 solution, 0 failures
The Big Picture
This experiment demonstrates several key aspects of using MoveIt Task Constructor for pick and place operations:
- Flexibility: The planner found multiple solutions for most stages, indicating its ability to handle various scenarios and constraints.
- Robustness: Despite some failures in individual substages (e.g., IK solutions), the overall task planning was successful, showcasing the planner’s ability to overcome local challenges.
- Complexity Handling: The planner effectively broke down the complex pick and place task into manageable stages, handling aspects like collision checking, IK solving, and Cartesian path planning.
- Efficiency: The hierarchical approach allowed for efficient planning of each stage while maintaining the overall task coherence.
- Detailed Feedback: The output provides a wealth of information about each stage and substage, allowing for deep analysis and potential optimization of the task.
By structuring our pick and place task this way, we achieve a balance of comprehensiveness and flexibility. The detailed stage breakdown allows for precise control and understanding of each part of the task, while the overall planning ensures that all stages work together seamlessly. This approach demonstrates the power of the MoveIt Task Constructor in handling complex manipulation tasks in robotics.
Detailed Code Walkthrough
pick_place_task.h
File Header and Includes
The file begins with a comprehensive comment block explaining the purpose of the file: defining the PickPlaceTask class for a pick and place demo using the MoveIt Task Constructor. It includes the necessary headers for ROS 2, MoveIt, and the MoveIt Task Constructor, setting up the foundation for our pick and place task.
The includes are grouped logically:
- ROS 2 headers
- MoveIt headers
- MoveIt Task Constructor headers
- Custom parameter header
Namespace and Using Directive
The code is encapsulated in the hello_moveit_task_constructor namespace, and it uses the moveit::task_constructor namespace for convenience.
setupDemoScene Function
This function is declared to set up the demo scene based on the provided parameters. It’s implemented in the corresponding .cpp file. The function takes a const reference to pick_place_demo::Params, which likely contains the configuration for the demo scene.
printStageDetails Function
This function is declared to print detailed information about a stage and its substages. It’s a recursive function that helps in debugging and understanding the task structure. It takes a pointer to a Stage object and an optional indent parameter for formatting the output.
PickPlaceTask Class
This class encapsulates the functionality for the pick and place task:
- Constructor: Takes a task name as input.
- Destructor: Default implementation.
- init: Initializes the task with the given node and parameters.
- plan: Plans the task, generating up to max_solutions solutions.
- execute: Executes the planned task.
The class has two private members:
- task_name_: Stores the name of the task.
- task_: A shared pointer to the MoveIt Task Constructor task.
This header file provides a clear structure for implementing a pick and place task using MoveIt Task Constructor. It separates the task setup (init), planning (plan), and execution (execute) into distinct methods, allowing for a modular approach to task construction and execution.
The use of parameters (pick_place_demo::Params) means the task is configurable, enhancing its reusability for different pick and place scenarios.
pick_place_demo.cpp
File Header and Includes
The file begins with a comprehensive comment block explaining the purpose of the file: to serve as the main entry point for the pick and place demo using MoveIt Task Constructor. It includes the necessary headers for ROS 2 and the custom pick_place_task implementation.
Logger Setup
A logger is set up for the demo, which is used for outputting information during the execution of the program.
Main Function
The main function is the entry point of the program.
ROS 2 Initialization and Node Setup
ROS 2 is initialized with the provided command-line arguments. Node options are configured to automatically declare parameters from overrides (launch files or yaml files). A ROS 2 node named “pick_place_demo” is created with these options.
Spinning Thread
A separate thread is created to handle ROS 2 callbacks, allowing the node to process incoming messages and services while the main thread continues execution.
Parameter Handling
A parameter listener is created to get the demo parameters, and the parameters are retrieved using the get_params() method.
Demo Scene Setup
The demo scene is set up based on the retrieved parameters using the setupDemoScene function.
Task Creation and Initialization
An instance of PickPlaceTask named “pick_place_task” is created. The task is initialized with the node and parameters. If initialization fails, the program logs an error and exits.
Task Planning and Execution
The program attempts to plan the pick and place task with the specified maximum number of solutions.
- If planning succeeds, it logs a success message.
- If execution is enabled in the parameters, it executes the plan and logs completion.
- If execution is disabled, it logs that execution is disabled.
- If planning fails, it logs a failure message.
Thread Joining
The program waits for the ROS 2 spinning thread to finish before exiting. This keeps the node alive for introspection.
pick_place_task.cpp
File Header and Includes
The file begins with a comprehensive comment block explaining the purpose of the file: implementing the PickPlaceTask class for a pick and place operation using the MoveIt Task Constructor. It includes necessary headers for Eigen geometry, ROS 2 messages, and TF2 transformations.
Namespace and Helper Functions
The code defines a unnamed namespace with two helper functions:
- vectorToEigen: Transforms a vector of doubles into a 3D position and orientation using Eigen.
- vectorToPose: Converts a vector of doubles to a geometry_msgs::msg::Pose.
These functions are used throughout the code to convert between different representation formats.
hello_moveit_task_constructor Namespace
The main implementation is within this namespace. It includes several functions.
spawnObject Function
This function adds a collision object to the planning scene.
createTable and createObject Functions
These functions create collision objects for the table and the object to be manipulated, respectively. They use the parameters provided to set up the geometry and pose of these objects.
setupDemoScene Function
This function sets up the demo scene by spawning the table (if specified) and the object to be manipulated.
printStageDetails Function
This function recursively prints detailed information about each stage in the task, including solutions, failures, and sub-stages for container stages.
PickPlaceTask Class Implementation
Constructor
A simple constructor that initializes the task name.
init Function
A complex function that sets up the entire pick and place task. It includes:
- Task initialization and property setting
- Creation of various planners (OMPL, Joint Interpolation, Cartesian)
- Setting up stages for the task, including:
- Current State
- Open Gripper
- Move to Pick
- Pick Object (which includes several sub-stages)
- Move to Place
- Place Object (which includes several sub-stages)
- Move to Home
Each stage is carefully configured with appropriate planners, properties, and constraints.
plan Function
This function attempts to plan the task. It logs the results, publishes solutions for visualization, and provides detailed stage summaries whether planning succeeds or fails.
execute Function
This function executes the planned task. It selects the first available solution and attempts to execute it, providing detailed logging of the execution process and any potential failures.
That’s it. In the next section, we will implement the exact same pick and place task using a single source code file rather than multiple files.
Example 2
Create the Code
Let’s go through another way of implementing a pick and place task with the MoveIt Task Constructor.
Open a new terminal window, and type:
cd ~/ros2_ws/src/mycobot_ros2/hello_moveit_task_constructor/src/
gedit mtc_node.cpp
Add this code.
/**
* @file mtc_node.cpp
* @brief Implementation of a MoveIt Task Constructor (MTC) node for a pick and place task.
*
* This program implements a pick and place task using MoveIt Task Constructor (MTC).
* It creates a planning scene, generates a series of motion stages, and executes them
* to pick up an object from one location and place it in another.
*
* @author Addison Sears-Collins
* @date August 26, 2024
*/
// Include necessary ROS 2 and MoveIt headers
#include <rclcpp/rclcpp.hpp>
#include <moveit/planning_scene/planning_scene.h>
#include <moveit/planning_scene_interface/planning_scene_interface.h>
#include <moveit/task_constructor/task.h>
#include <moveit/task_constructor/solvers.h>
#include <moveit/task_constructor/stages.h>
// Other utilities
#include <type_traits>
#include <string>
#include <vector>
// Conditional includes for tf2 geometry messages and Eigen
#include <Eigen/Geometry>
#include <geometry_msgs/msg/pose.hpp>
#if __has_include(<tf2_geometry_msgs/tf2_geometry_msgs.hpp>)
#include <tf2_geometry_msgs/tf2_geometry_msgs.hpp>
#else
#include <tf2_geometry_msgs/tf2_geometry_msgs.h>
#endif
#if __has_include(<tf2_eigen/tf2_eigen.hpp>)
#include <tf2_eigen/tf2_eigen.hpp>
#else
#include <tf2_eigen/tf2_eigen.h>
#endif
namespace {
/**
* @brief Transform a vector of numbers into a 3D position and orientation.
* @param values Vector containing position and orientation values.
* @return Eigen::Isometry3d representing the transformation.
*/
Eigen::Isometry3d vectorToEigen(const std::vector<double>& values) {
return Eigen::Translation3d(values[0], values[1], values[2]) *
Eigen::AngleAxisd(values[3], Eigen::Vector3d::UnitX()) *
Eigen::AngleAxisd(values[4], Eigen::Vector3d::UnitY()) *
Eigen::AngleAxisd(values[5], Eigen::Vector3d::UnitZ());
}
/**
* @brief Convert a vector of numbers to a geometry_msgs::msg::Pose.
* @param values Vector containing position and orientation values.
* @return geometry_msgs::msg::Pose representing the pose.
*/
geometry_msgs::msg::Pose vectorToPose(const std::vector<double>& values) {
return tf2::toMsg(vectorToEigen(values));
};
} // namespace
// Namespace alias for MoveIt Task Constructor
namespace mtc = moveit::task_constructor;
/**
* @brief Class representing the MTC Task Node.
*/
class MTCTaskNode : public rclcpp::Node
{
public:
MTCTaskNode(const rclcpp::NodeOptions& options);
void doTask();
void setupPlanningScene();
private:
mtc::Task task_;
mtc::Task createTask();
};
/**
* @brief Constructor for the MTCTaskNode class.
* @param options Node options for configuration.
*/
MTCTaskNode::MTCTaskNode(const rclcpp::NodeOptions& options)
: Node("mtc_node", options)
{
auto declare_parameter = [this](const std::string& name, const auto& default_value, const std::string& description = "") {
rcl_interfaces::msg::ParameterDescriptor descriptor;
descriptor.description = description;
if (!this->has_parameter(name)) {
this->declare_parameter(name, default_value, descriptor);
}
};
// General parameters
declare_parameter("execute", false, "Whether to execute the planned task");
declare_parameter("max_solutions", 10, "Maximum number of solutions to compute");
declare_parameter("spawn_table", true, "Whether to spawn a table in the planning scene");
// Controller parameters
declare_parameter("controller_names", std::vector<std::string>{"arm_controller", "grip_action_controller"}, "Names of the controllers to use");
// Robot configuration parameters
declare_parameter("arm_group_name", "arm", "Name of the arm group in the SRDF");
declare_parameter("gripper_group_name", "gripper", "Name of the gripper group in the SRDF");
declare_parameter("gripper_frame", "link6_flange", "Name of the gripper frame");
declare_parameter("gripper_open_pose", "open", "Name of the gripper open pose");
declare_parameter("gripper_close_pose", "half_closed", "Name of the gripper closed pose");
declare_parameter("arm_home_pose", "home", "Name of the arm home pose");
// Scene frame parameters
declare_parameter("world_frame", "base_link", "Name of the world frame");
// Table parameters
declare_parameter("table_name", "table", "Name of the table in the planning scene");
declare_parameter("table_reference_frame", "base_link", "Reference frame for the table");
declare_parameter("table_dimensions", std::vector<double>{0.10, 0.20, 0.03}, "Dimensions of the table [x, y, z]");
declare_parameter("table_pose", std::vector<double>{0.22, 0.12, 0.0, 0.0, 0.0, 0.0}, "Pose of the table [x, y, z, roll, pitch, yaw]");
// Object parameters
declare_parameter("object_name", "object", "Name of the object to be manipulated");
declare_parameter("object_reference_frame", "base_link", "Reference frame for the object");
declare_parameter("object_dimensions", std::vector<double>{0.35, 0.0125}, "Dimensions of the object [height, radius]");
declare_parameter("object_pose", std::vector<double>{0.22, 0.12, 0.0, 0.0, 0.0, 0.0}, "Initial pose of the object [x, y, z, roll, pitch, yaw]");
// Grasp and place parameters
declare_parameter("grasp_frame_transform", std::vector<double>{0.0, 0.0, 0.096, 1.5708, 0.0, 0.0}, "Transform from gripper frame to grasp frame [x, y, z, roll, pitch, yaw]");
declare_parameter("place_pose", std::vector<double>{-0.183, -0.14, 0.0, 0.0, 0.0, 0.0}, "Pose where the object should be placed [x, y, z, roll, pitch, yaw]");
declare_parameter("place_surface_offset", -0.03, "Offset from the surface when placing the object");
// Motion planning parameters
declare_parameter("approach_object_min_dist", 0.0015, "Minimum approach distance to the object");
declare_parameter("approach_object_max_dist", 0.3, "Maximum approach distance to the object");
declare_parameter("lift_object_min_dist", 0.005, "Minimum lift distance for the object");
declare_parameter("lift_object_max_dist", 0.3, "Maximum lift distance for the object");
declare_parameter("lower_object_min_dist", 0.005, "Minimum distance for lowering object");
declare_parameter("lower_object_max_dist", 0.4, "Maximum distance for lowering object");
// Timeout parameters
declare_parameter("move_to_pick_timeout", 5.0, "Timeout for move to pick stage (seconds)");
declare_parameter("move_to_place_timeout", 10.0, "Timeout for move to place stage (seconds)");
// Grasp generation parameters
declare_parameter("grasp_pose_angle_delta", 0.2618, "Angular resolution for sampling grasp poses (radians)");
declare_parameter("grasp_pose_max_ik_solutions", 8, "Maximum number of IK solutions for grasp pose generation");
declare_parameter("grasp_pose_min_solution_distance", 1.0, "Minimum distance in joint-space units between IK solutions for grasp pose");
// Place generation parameters
declare_parameter("place_pose_max_ik_solutions", 2, "Maximum number of IK solutions for place pose generation");
// Cartesian planner parameters
declare_parameter("cartesian_max_velocity_scaling", 1.0, "Max velocity scaling factor for Cartesian planner");
declare_parameter("cartesian_max_acceleration_scaling", 1.0, "Max acceleration scaling factor for Cartesian planner");
declare_parameter("cartesian_step_size", 0.00025, "Step size for Cartesian planner");
// Direction vector parameters
declare_parameter("approach_object_direction_z", 1.0, "Z component of approach object direction vector");
declare_parameter("lift_object_direction_z", 1.0, "Z component of lift object direction vector");
declare_parameter("lower_object_direction_z", -1.0, "Z component of lower object direction vector");
declare_parameter("retreat_direction_z", -1.0, "Z component of retreat direction vector");
// Other parameters
declare_parameter("place_pose_z_offset_factor", 0.5, "Factor to multiply object height for place pose Z offset");
declare_parameter("retreat_min_distance", 0.025, "Minimum distance for retreat motion");
declare_parameter("retreat_max_distance", 0.25, "Maximum distance for retreat motion");
RCLCPP_INFO(this->get_logger(), "All parameters have been declared with descriptions");
}
/**
* @brief Set up the planning scene with collision objects.
*/
void MTCTaskNode::setupPlanningScene()
{
// Create a planning scene interface to interact with the world
moveit::planning_interface::PlanningSceneInterface psi;
// Get general parameters
auto spawn_table = this->get_parameter("spawn_table").as_bool();
// Get table parameters
auto table_name = this->get_parameter("table_name").as_string();
auto table_dimensions = this->get_parameter("table_dimensions").as_double_array();
auto table_pose_param = this->get_parameter("table_pose").as_double_array();
auto table_reference_frame = this->get_parameter("table_reference_frame").as_string();
if (spawn_table) {
// Create a table collision object
geometry_msgs::msg::Pose table_pose = vectorToPose(table_pose_param);
moveit_msgs::msg::CollisionObject table_object;
table_object.id = table_name;
table_object.header.frame_id = table_reference_frame;
table_object.primitives.resize(1);
table_object.primitives[0].type = shape_msgs::msg::SolidPrimitive::BOX;
table_object.primitives[0].dimensions = { table_dimensions.at(0), table_dimensions.at(1),
table_dimensions.at(2) };
table_pose.position.z -= 0.5 * table_dimensions[2]; // align surface with world
table_object.primitive_poses.push_back(table_pose);
// Add the table to the planning scene
if (!psi.applyCollisionObject(table_object)) {
RCLCPP_ERROR(this->get_logger(), "Failed to spawn table object: %s", table_object.id.c_str());
throw std::runtime_error("Failed to spawn table object: " + table_object.id);
}
RCLCPP_INFO(this->get_logger(), "Added table to planning scene");
} else {
RCLCPP_INFO(this->get_logger(), "Skipping table spawn as per configuration");
}
// Get object parameters
auto object_name = this->get_parameter("object_name").as_string();
auto object_dimensions = this->get_parameter("object_dimensions").as_double_array();
auto object_pose_param = this->get_parameter("object_pose").as_double_array();
auto object_reference_frame = this->get_parameter("object_reference_frame").as_string();
// Create a cylinder collision object
geometry_msgs::msg::Pose cylinder_pose = vectorToPose(object_pose_param);
auto place_pose_z_offset_factor = this->get_parameter("place_pose_z_offset_factor").as_double();
cylinder_pose.position.z += place_pose_z_offset_factor * object_dimensions[0]; // Adjust z position before creating the object
moveit_msgs::msg::CollisionObject cylinder_object;
cylinder_object.id = object_name;
cylinder_object.header.frame_id = object_reference_frame;
cylinder_object.primitives.resize(1);
cylinder_object.primitives[0].type = shape_msgs::msg::SolidPrimitive::CYLINDER;
cylinder_object.primitives[0].dimensions = { object_dimensions.at(0), object_dimensions.at(1) };
cylinder_object.primitive_poses.push_back(cylinder_pose);
// Add the cylinder to the planning scene
if (!psi.applyCollisionObject(cylinder_object)) {
RCLCPP_ERROR(this->get_logger(), "Failed to spawn object: %s", cylinder_object.id.c_str());
throw std::runtime_error("Failed to spawn object: " + cylinder_object.id);
}
RCLCPP_INFO(this->get_logger(), "Added object to planning scene");
RCLCPP_INFO(this->get_logger(), "Planning scene setup completed");
}
/**
* @brief Plan and/or execute the pick and place task.
*/
void MTCTaskNode::doTask()
{
RCLCPP_INFO(this->get_logger(), "Starting the pick and place task");
task_ = createTask();
// Get parameters
auto execute = this->get_parameter("execute").as_bool();
auto max_solutions = this->get_parameter("max_solutions").as_int();
try
{
task_.init();
RCLCPP_INFO(this->get_logger(), "Task initialized successfully");
}
catch (mtc::InitStageException& e)
{
RCLCPP_ERROR(this->get_logger(), "Task initialization failed: %s", e.what());
return;
}
// Attempt to plan the task
if (!task_.plan(max_solutions))
{
RCLCPP_ERROR(this->get_logger(), "Task planning failed");
return;
}
RCLCPP_INFO(this->get_logger(), "Task planning succeeded");
// Publish the planned solution for visualization
task_.introspection().publishSolution(*task_.solutions().front());
RCLCPP_INFO(this->get_logger(), "Published solution for visualization");
if (execute)
{
// Execute the planned task
RCLCPP_INFO(this->get_logger(), "Executing the planned task");
auto result = task_.execute(*task_.solutions().front());
if (result.val != moveit_msgs::msg::MoveItErrorCodes::SUCCESS)
{
RCLCPP_ERROR(this->get_logger(), "Task execution failed with error code: %d", result.val);
return;
}
RCLCPP_INFO(this->get_logger(), "Task executed successfully");
}
else
{
RCLCPP_INFO(this->get_logger(), "Execution skipped as per configuration");
}
return;
}
/**
* @brief Create the MTC task with all necessary stages.
* @return The created MTC task.
*/
mtc::Task MTCTaskNode::createTask()
{
RCLCPP_INFO(this->get_logger(), "Creating MTC task");
// Create a new Task
mtc::Task task;
// Set the name of the task
task.stages()->setName("pick_place_task");
// Load the robot model into the task
task.loadRobotModel(shared_from_this(), "robot_description");
// Get parameters
// Robot configuration parameters
auto arm_group_name = this->get_parameter("arm_group_name").as_string();
auto gripper_group_name = this->get_parameter("gripper_group_name").as_string();
auto gripper_frame = this->get_parameter("gripper_frame").as_string();
auto arm_home_pose = this->get_parameter("arm_home_pose").as_string();
// Gripper poses
auto gripper_open_pose = this->get_parameter("gripper_open_pose").as_string();
auto gripper_close_pose = this->get_parameter("gripper_close_pose").as_string();
// Frame parameters
auto world_frame = this->get_parameter("world_frame").as_string();
// Controller parameters
auto controller_names = this->get_parameter("controller_names").as_string_array();
// Object parameters
auto object_name = this->get_parameter("object_name").as_string();
auto object_reference_frame = this->get_parameter("object_reference_frame").as_string();
auto object_dimensions = this->get_parameter("object_dimensions").as_double_array();
auto object_pose = this->get_parameter("object_pose").as_double_array();
// Table parameters
auto table_name = this->get_parameter("table_name").as_string();
auto table_reference_frame = this->get_parameter("table_reference_frame").as_string();
// Grasp and place parameters
auto grasp_frame_transform = this->get_parameter("grasp_frame_transform").as_double_array();
auto place_pose = this->get_parameter("place_pose").as_double_array();
auto place_surface_offset = this->get_parameter("place_surface_offset").as_double();
// Motion planning parameters
auto approach_object_min_dist = this->get_parameter("approach_object_min_dist").as_double();
auto approach_object_max_dist = this->get_parameter("approach_object_max_dist").as_double();
auto lift_object_min_dist = this->get_parameter("lift_object_min_dist").as_double();
auto lift_object_max_dist = this->get_parameter("lift_object_max_dist").as_double();
auto lower_object_min_dist = this->get_parameter("lower_object_min_dist").as_double();
auto lower_object_max_dist = this->get_parameter("lower_object_max_dist").as_double();
// Timeout parameters
auto move_to_pick_timeout = this->get_parameter("move_to_pick_timeout").as_double();
auto move_to_place_timeout = this->get_parameter("move_to_place_timeout").as_double();
// Grasp generation parameters
auto grasp_pose_angle_delta = this->get_parameter("grasp_pose_angle_delta").as_double();
auto grasp_pose_max_ik_solutions = this->get_parameter("grasp_pose_max_ik_solutions").as_int();
auto grasp_pose_min_solution_distance = this->get_parameter("grasp_pose_min_solution_distance").as_double();
// Place generation parameters
auto place_pose_max_ik_solutions = this->get_parameter("place_pose_max_ik_solutions").as_int();
// Cartesian planner parameters
auto cartesian_max_velocity_scaling = this->get_parameter("cartesian_max_velocity_scaling").as_double();
auto cartesian_max_acceleration_scaling = this->get_parameter("cartesian_max_acceleration_scaling").as_double();
auto cartesian_step_size = this->get_parameter("cartesian_step_size").as_double();
// Direction vector parameters
auto approach_object_direction_z = this->get_parameter("approach_object_direction_z").as_double();
auto lift_object_direction_z = this->get_parameter("lift_object_direction_z").as_double();
auto lower_object_direction_z = this->get_parameter("lower_object_direction_z").as_double();
auto retreat_direction_z = this->get_parameter("retreat_direction_z").as_double();
// Other parameters
auto place_pose_z_offset_factor = this->get_parameter("place_pose_z_offset_factor").as_double();
auto retreat_min_distance = this->get_parameter("retreat_min_distance").as_double();
auto retreat_max_distance = this->get_parameter("retreat_max_distance").as_double();
// Create planners for different types of motion
// Pipeline planner for complex movements
// OMPL planner
std::unordered_map<std::string, std::string> ompl_map_arm = {
{"ompl", arm_group_name + "[RRTConnectkConfigDefault]"}
};
auto ompl_planner_arm = std::make_shared<mtc::solvers::PipelinePlanner>(
this->shared_from_this(),
ompl_map_arm);
RCLCPP_INFO(this->get_logger(), "OMPL planner created for the arm group");
// JointInterpolation is a basic planner that is used for simple motions
// It computes quickly but doesn't support complex motions.
auto interpolation_planner = std::make_shared<mtc::solvers::JointInterpolationPlanner>();
RCLCPP_INFO(this->get_logger(), "Joint Interpolation planner created for the gripper group");
// Cartesian planner
auto cartesian_planner = std::make_shared<mtc::solvers::CartesianPath>();
cartesian_planner->setMaxVelocityScalingFactor(cartesian_max_velocity_scaling);
cartesian_planner->setMaxAccelerationScalingFactor(cartesian_max_acceleration_scaling);
cartesian_planner->setStepSize(cartesian_step_size);
RCLCPP_INFO(this->get_logger(), "Cartesian planner created");
// Set task properties
task.setProperty("trajectory_execution_info",
mtc::TrajectoryExecutionInfo().set__controller_names(controller_names));
task.setProperty("group", arm_group_name); // The main planning group
task.setProperty("eef", gripper_group_name); // The end-effector group
task.setProperty("ik_frame", gripper_frame); // The frame for inverse kinematics
/****************************************************
* *
* Current State *
* *
***************************************************/
// Pointer to store the current state (will be used during the grasp pose generation stage)
mtc::Stage* current_state_ptr = nullptr;
// Add a stage to capture the current state
auto stage_state_current = std::make_unique<mtc::stages::CurrentState>("current state");
current_state_ptr = stage_state_current.get();
task.add(std::move(stage_state_current));
/****************************************************
* *
* Open Gripper *
* *
***************************************************/
// This stage is responsible for opening the robot's gripper in preparation for picking
// up an object in the pick-and-place task.
auto stage_open_gripper =
std::make_unique<mtc::stages::MoveTo>("open gripper", interpolation_planner);
stage_open_gripper->setGroup(gripper_group_name);
stage_open_gripper->setGoal(gripper_open_pose);
stage_open_gripper->properties().set("trajectory_execution_info",
mtc::TrajectoryExecutionInfo().set__controller_names(controller_names));
task.add(std::move(stage_open_gripper));
/****************************************************
* *
* Move to Pick *
* *
***************************************************/
// Create a stage to move the arm to a pre-grasp position
auto stage_move_to_pick = std::make_unique<mtc::stages::Connect>(
"move to pick",
mtc::stages::Connect::GroupPlannerVector{
{arm_group_name, ompl_planner_arm},
{gripper_group_name, interpolation_planner}
});
stage_move_to_pick->setTimeout(move_to_pick_timeout);
stage_move_to_pick->properties().configureInitFrom(mtc::Stage::PARENT);
task.add(std::move(stage_move_to_pick));
// Create a pointer for the stage that will attach the object (to be used later)
// By declaring it at the top level of the function, it can be accessed throughout
// the entire task creation process.
// This allows different parts of the code to use and modify this pointer.
mtc::Stage* attach_object_stage =
nullptr; // Forward attach_object_stage to place pose generator
/****************************************************
* *
* Pick Object *
* *
***************************************************/
{
// Create a serial container for the grasping action
// This container will hold stages (in order) that will accomplish the picking action
auto grasp = std::make_unique<mtc::SerialContainer>("pick object");
task.properties().exposeTo(grasp->properties(), { "eef", "group", "ik_frame" });
grasp->properties().configureInitFrom(mtc::Stage::PARENT,
{ "eef", "group", "ik_frame" });
/****************************************************
---- * Approach Object *
***************************************************/
{
// Create a stage for moving the gripper close to the object before trying to grab it.
// We are doing a movement that is relative to our current position.
// Cartesian planner will move the gripper in a straight line
auto stage =
std::make_unique<mtc::stages::MoveRelative>("approach object", cartesian_planner);
// Set properties for visualization and planning
stage->properties().set("marker_ns", "approach_object"); // Namespace for visualization markers
stage->properties().set("link", gripper_frame); // The link to move (end effector)
stage->properties().set("trajectory_execution_info",
mtc::TrajectoryExecutionInfo().set__controller_names(controller_names));
stage->properties().configureInitFrom(mtc::Stage::PARENT, { "group" }); // Inherit the 'group' property
stage->setMinMaxDistance(approach_object_min_dist, approach_object_max_dist);
// Define the direction that we want the gripper to move (i.e. z direction) from the gripper frame
geometry_msgs::msg::Vector3Stamped vec;
vec.header.frame_id = gripper_frame; // Set the frame for the vector
vec.vector.z = approach_object_direction_z; // Set the direction (in this case, along the z-axis of the gripper frame)
stage->setDirection(vec);
grasp->insert(std::move(stage));
}
/****************************************************
---- * Generate Grasp Pose *
***************************************************/
{
// Generate the grasp pose
// This is the stage for computing how the robot should grab the object
// This stage is a generator stage because it doesn't need information from
// stages before or after it.
// When generating solutions, MTC will try to grab the object from many different orientations.
// Sample grasp pose candidates in angle increments around the z-axis of the object
auto stage = std::make_unique<mtc::stages::GenerateGraspPose>("generate grasp pose");
stage->properties().configureInitFrom(mtc::Stage::PARENT);
stage->properties().set("marker_ns", "grasp_pose");
stage->setPreGraspPose(gripper_open_pose);
stage->setObject(object_name);
stage->setAngleDelta(grasp_pose_angle_delta); // Angular resolution for sampling grasp poses around the object
stage->setMonitoredStage(current_state_ptr); // Ensure grasp poses are valid given the initial configuration of the robot
// Compute IK for sampled grasp poses
auto wrapper = std::make_unique<mtc::stages::ComputeIK>("grasp pose IK", std::move(stage));
wrapper->setMaxIKSolutions(grasp_pose_max_ik_solutions);
wrapper->setMinSolutionDistance(grasp_pose_min_solution_distance);
wrapper->setIKFrame(vectorToEigen(grasp_frame_transform), gripper_frame); // Transform from gripper frame to tool center point (TCP)
wrapper->properties().configureInitFrom(mtc::Stage::PARENT, { "eef", "group" });
wrapper->properties().configureInitFrom(mtc::Stage::INTERFACE, { "target_pose" });
grasp->insert(std::move(wrapper));
}
/****************************************************
---- * Allow Collision (gripper, object) *
***************************************************/
{
// Modify planning scene (w/o altering the robot's pose) to allow touching the object for picking
auto stage =
std::make_unique<mtc::stages::ModifyPlanningScene>("allow collision (gripper,object)");
stage->allowCollisions(
object_name,
task.getRobotModel()
->getJointModelGroup(gripper_group_name)
->getLinkModelNamesWithCollisionGeometry(),
true);
grasp->insert(std::move(stage));
}
/****************************************************
---- * Close Gripper *
***************************************************/
{
auto stage = std::make_unique<mtc::stages::MoveTo>("close gripper", interpolation_planner);
stage->setGroup(gripper_group_name);
stage->setGoal(gripper_close_pose);
stage->properties().set("trajectory_execution_info",
mtc::TrajectoryExecutionInfo().set__controller_names(controller_names));
grasp->insert(std::move(stage));
}
/****************************************************
---- * Attach Object *
***************************************************/
{
auto stage = std::make_unique<mtc::stages::ModifyPlanningScene>("attach object");
stage->attachObject(object_name, gripper_frame); // attach object to gripper_frame
attach_object_stage = stage.get();
grasp->insert(std::move(stage));
}
/****************************************************
---- * Allow collision (object, surface) *
***************************************************/
{
// Allows the planner to generate valid trajectories where the object remains in contact
// with the support surface until it's lifted.
auto stage = std::make_unique<mtc::stages::ModifyPlanningScene>("allow collision (object,support)");
stage->allowCollisions({ object_name }, {table_name}, true);
grasp->insert(std::move(stage));
}
/****************************************************
---- * Lift object *
***************************************************/
{
auto stage = std::make_unique<mtc::stages::MoveRelative>("lift object", cartesian_planner);
stage->properties().configureInitFrom(mtc::Stage::PARENT, { "group" });
stage->setMinMaxDistance(lift_object_min_dist, lift_object_max_dist);
stage->setIKFrame(gripper_frame);
stage->properties().set("marker_ns", "lift_object");
stage->properties().set("trajectory_execution_info",
mtc::TrajectoryExecutionInfo().set__controller_names(controller_names));
// We're defining the direction to lift the object
geometry_msgs::msg::Vector3Stamped vec;
vec.header.frame_id = world_frame;
vec.vector.z = lift_object_direction_z; // This means "straight up"
stage->setDirection(vec);
grasp->insert(std::move(stage));
}
/****************************************************
---- * Forbid collision (object, surface)* *
***************************************************/
{
// Forbid collisions between the picked object and the support surface.
// This is important after the object has been lifted to ensure it doesn't accidentally
// collide with the surface during subsequent movements.
auto stage = std::make_unique<mtc::stages::ModifyPlanningScene>("forbid collision (object,surface)");
stage->allowCollisions({ object_name }, {table_name}, false);
grasp->insert(std::move(stage));
}
// Add the serial container to the robot's to-do list
// This serial container contains all the sequential steps we've created for grasping
// and lifting the object
task.add(std::move(grasp));
}
/******************************************************
* *
* Move to Place *
* *
*****************************************************/
{
// Connect the grasped state to the pre-place state, i.e. realize the object transport
// In other words, this stage plans the motion that transports the object from where it was picked up
// to where it will be placed.
auto stage_move_to_place = std::make_unique<mtc::stages::Connect>(
"move to place",
mtc::stages::Connect::GroupPlannerVector{
{arm_group_name, ompl_planner_arm},
{gripper_group_name, interpolation_planner}
});
stage_move_to_place->setTimeout(move_to_place_timeout);
stage_move_to_place->properties().configureInitFrom(mtc::Stage::PARENT);
task.add(std::move(stage_move_to_place));
}
/******************************************************
* *
* Place Object *
* *
*****************************************************/
// All placing sub-stages are collected within a serial container
{
auto place = std::make_unique<mtc::SerialContainer>("place object");
task.properties().exposeTo(place->properties(), { "eef", "group", "ik_frame" });
place->properties().configureInitFrom(mtc::Stage::PARENT, { "eef", "group", "ik_frame" });
/******************************************************
---- * Lower Object *
*****************************************************/
{
auto stage = std::make_unique<mtc::stages::MoveRelative>("lower object", cartesian_planner);
stage->properties().set("marker_ns", "lower_object");
stage->properties().set("link", gripper_frame);
stage->properties().set("trajectory_execution_info",
mtc::TrajectoryExecutionInfo().set__controller_names(controller_names));
stage->properties().configureInitFrom(mtc::Stage::PARENT, { "group" });
stage->setMinMaxDistance(lower_object_min_dist, lower_object_max_dist);
// Set downward direction
geometry_msgs::msg::Vector3Stamped vec;
vec.header.frame_id = world_frame;
vec.vector.z = lower_object_direction_z;
stage->setDirection(vec);
place->insert(std::move(stage));
}
/******************************************************
---- * Generate Place Pose *
*****************************************************/
{
// Generate Place Pose
auto stage = std::make_unique<mtc::stages::GeneratePlacePose>("generate place pose");
stage->properties().configureInitFrom(mtc::Stage::PARENT, { "ik_frame" });
stage->properties().set("marker_ns", "place_pose");
stage->setObject(object_name);
// Set target pose
geometry_msgs::msg::PoseStamped target_pose_msg;
target_pose_msg.header.frame_id = world_frame;
target_pose_msg.pose = vectorToPose(place_pose);
target_pose_msg.pose.position.z += place_pose_z_offset_factor * object_dimensions[0] + place_surface_offset;
stage->setPose(target_pose_msg);
stage->setMonitoredStage(attach_object_stage); // hook into successful pick solutions
// Compute IK
auto wrapper = std::make_unique<mtc::stages::ComputeIK>("place pose IK", std::move(stage));
wrapper->setMaxIKSolutions(place_pose_max_ik_solutions);
wrapper->setIKFrame(vectorToEigen(grasp_frame_transform), gripper_frame); // Transform from gripper frame to tool center point (TCP)
wrapper->properties().configureInitFrom(mtc::Stage::PARENT, { "eef", "group" });
wrapper->properties().configureInitFrom(mtc::Stage::INTERFACE, { "target_pose" });
place->insert(std::move(wrapper));
}
/******************************************************
---- * Open Gripper *
*****************************************************/
{
auto stage = std::make_unique<mtc::stages::MoveTo>("open gripper", interpolation_planner);
stage->setGroup(gripper_group_name);
stage->setGoal(gripper_open_pose);
stage->properties().set("trajectory_execution_info",
mtc::TrajectoryExecutionInfo().set__controller_names(controller_names));
place->insert(std::move(stage));
}
/******************************************************
---- * Forbid collision (gripper, object) *
*****************************************************/
{
auto stage = std::make_unique<mtc::stages::ModifyPlanningScene>("forbid collision (gripper,object)");
stage->allowCollisions(object_name, *task.getRobotModel()->getJointModelGroup(gripper_group_name),
false);
place->insert(std::move(stage));
}
/******************************************************
---- * Detach Object *
*****************************************************/
{
// Update the planning scene to reflect that the object is no longer attached to the gripper.
auto stage = std::make_unique<mtc::stages::ModifyPlanningScene>("detach object");
stage->detachObject(object_name, gripper_frame);
place->insert(std::move(stage));
}
/******************************************************
---- * Retreat Motion *
*****************************************************/
{
auto stage = std::make_unique<mtc::stages::MoveRelative>("retreat after place", cartesian_planner);
stage->properties().set("trajectory_execution_info",
mtc::TrajectoryExecutionInfo().set__controller_names(controller_names));
stage->properties().configureInitFrom(mtc::Stage::PARENT, { "group" });
stage->setMinMaxDistance(retreat_min_distance, retreat_max_distance);
stage->setIKFrame(gripper_frame);
stage->properties().set("marker_ns", "retreat");
geometry_msgs::msg::Vector3Stamped vec;
vec.header.frame_id = gripper_frame;
vec.vector.z = retreat_direction_z;
stage->setDirection(vec);
place->insert(std::move(stage));
}
// Add place container to task
task.add(std::move(place));
}
/******************************************************
* *
* Move to Home *
* *
*****************************************************/
{
auto stage = std::make_unique<mtc::stages::MoveTo>("move home", ompl_planner_arm);
stage->properties().set("trajectory_execution_info",
mtc::TrajectoryExecutionInfo().set__controller_names(controller_names));
stage->properties().configureInitFrom(mtc::Stage::PARENT, { "group" });
stage->setGoal(arm_home_pose);
task.add(std::move(stage));
}
return task;
}
/**
* @brief Main function to run the MTC task node.
* @param argc Number of command-line arguments.
* @param argv Array of command-line arguments.
* @return Exit status.
*/
int main(int argc, char** argv)
{
// Initialize ROS 2
rclcpp::init(argc, argv);
// Set up node options
rclcpp::NodeOptions options;
options.automatically_declare_parameters_from_overrides(true);
// Create the MTC task node
auto mtc_task_node = std::make_shared<MTCTaskNode>(options);
// Set up a multi-threaded executor
rclcpp::executors::MultiThreadedExecutor executor;
executor.add_node(mtc_task_node);
// Set up the planning scene and execute the task
try {
RCLCPP_INFO(mtc_task_node->get_logger(), "Setting up planning scene");
mtc_task_node->setupPlanningScene();
RCLCPP_INFO(mtc_task_node->get_logger(), "Executing task");
mtc_task_node->doTask();
RCLCPP_INFO(mtc_task_node->get_logger(), "Task execution completed. Keeping node alive for visualization. Press Ctrl+C to exit.");
} catch (const std::exception& e) {
RCLCPP_ERROR(mtc_task_node->get_logger(), "An error occurred: %s", e.what());
}
// Keep the node running until Ctrl+C is pressed
executor.spin();
// Cleanup
rclcpp::shutdown();
return 0;
}
Save the file, and close it.
Create a Parameter File
Open a new terminal window, and type:
cd ~/ros2_ws/src/mycobot_ros2/hello_moveit_task_constructor/config/
gedit mtc_node_params.yaml
Add this code.
mtc_node:
ros__parameters:
# General parameters
execute: false
max_solutions: 10
spawn_table: true
# Controller parameters
controller_names:
- "arm_controller"
- "grip_action_controller"
# Robot configuration parameters
arm_group_name: "arm"
gripper_group_name: "gripper"
gripper_frame: "link6_flange"
gripper_open_pose: "open"
gripper_close_pose: "half_closed"
arm_home_pose: "home"
# Scene frame parameters
world_frame: "base_link"
# Table parameters
table_name: "table"
table_reference_frame: "base_link"
table_dimensions: [0.10, 0.20, 0.03]
table_pose: [0.22, 0.12, 0.0, 0.0, 0.0, 0.0]
# Object parameters
object_name: "object"
object_reference_frame: "base_link"
object_dimensions: [0.35, 0.0125]
object_pose: [0.22, 0.12, 0.0, 0.0, 0.0, 0.0]
# Grasp and place parameters
grasp_frame_transform: [0.0, 0.0, 0.096, 1.5708, 0.0, 0.0]
place_pose: [-0.183, -0.14, 0.0, 0.0, 0.0, 0.0]
place_surface_offset: -0.03
# Motion planning parameters
approach_object_min_dist: 0.0015
approach_object_max_dist: 0.3
lift_object_min_dist: 0.005
lift_object_max_dist: 0.3
lower_object_min_dist: 0.005
lower_object_max_dist: 0.4
# Timeout parameters
move_to_pick_timeout: 5.0
move_to_place_timeout: 10.0
# Grasp generation parameters
grasp_pose_angle_delta: 0.2618 # Approximately pi/12 in radians
grasp_pose_max_ik_solutions: 8
grasp_pose_min_solution_distance: 1.0
# Place generation parameters
place_pose_max_ik_solutions: 2
# Cartesian planner parameters
cartesian_max_velocity_scaling: 1.0
cartesian_max_acceleration_scaling: 1.0
cartesian_step_size: 0.00025
# Direction vector parameters
approach_object_direction_z: 1.0
lift_object_direction_z: 1.0
lower_object_direction_z: -1.0
retreat_direction_z: -1.0
# Other parameters
place_pose_z_offset_factor: 0.5
retreat_min_distance: 0.025
retreat_max_distance: 0.25
Save the file, and close it.
Build the Code
cd ~/ros2_ws/
colcon build
source ~/.bashrc
Launch
Run the following commands to launch our standard MoveIt 2 environment:
ros2 launch mycobot_gazebo mycobot_280_arduino_bringup_ros2_control_gazebo.launch.py use_rviz:=false
ros2 launch hello_moveit_task_constructor demo.launch.py
Let’s run the mtc_node which has our pick and place task.
ros2 launch hello_moveit_task_constructor run.launch.py exe:=mtc_node
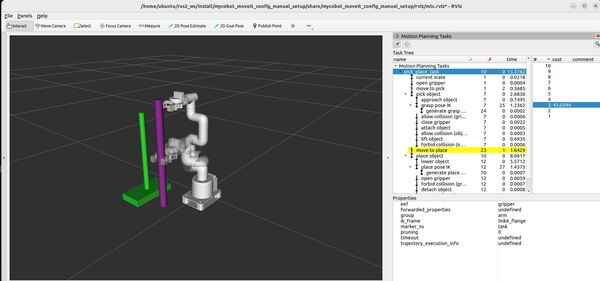
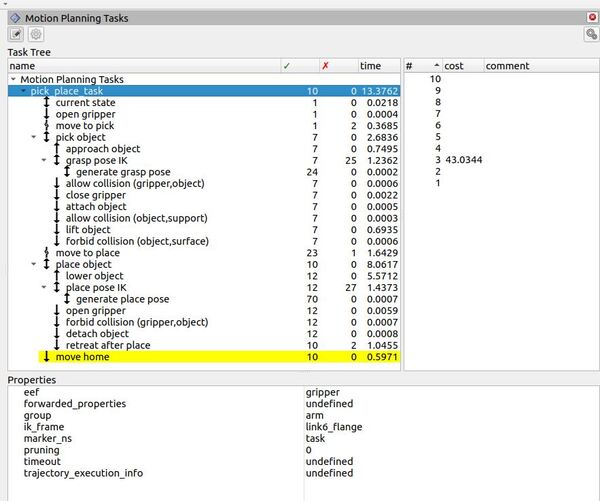
Change execute to true in the parameters file, and then build the package, and relaunch the mtc_node to make the robot execute the plan.
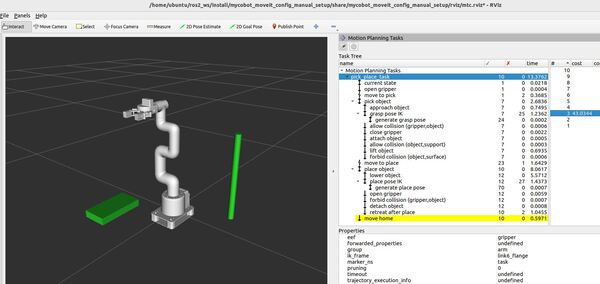
Detailed Code Walkthrough
Let’s go through each piece of mtc_node.cpp, step by step.
File Header and Includes
The file begins with a comprehensive comment block that outlines its purpose. It explains that this file implements a pick and place task using the MoveIt Task Constructor (MTC). The header provides context about what the program does, creating a planning scene and generating a series of motion stages to pick up an object and place it elsewhere.
Following the header, the code includes necessary headers for ROS 2, MoveIt, and the Task Constructor library. These includes bring in the required components for robot motion planning, scene manipulation, and task construction.
The inclusion of these headers indicates that this code will be working with ROS 2 for robot control, MoveIt for motion planning, and the Task Constructor for creating complex, multi-stage motion plans.
Utility Functions
The code defines two utility functions: vectorToEigen and vectorToPose. These functions convert vectors of doubles to Eigen transformations and geometry messages, respectively.
The vectorToEigen function takes a vector of 6 values (x, y, z, roll, pitch, yaw) and converts it into an Eigen::Isometry3d, which represents a 3D transformation.
The vectorToPose function uses vectorToEigen and converts the result to a geometry_msgs::msg::Pose, which is a ROS message type representing a position and orientation in 3D space.
These utility functions are used throughout the code to simplify the process of creating poses and transformations, making the code more readable and reducing the chance of errors in these conversions.
MTCTaskNode Class
The main class MTCTaskNode is defined, inheriting from rclcpp::Node. This class encapsulates the functionality for the MTC task. It declares public methods for executing the task (doTask) and setting up the planning scene (setupPlanningScene), as well as a private method for creating the task (createTask) and a private member to store the task (task_). This class structure follows object-oriented design principles, encapsulating related functionality and data within a single class.
MTCTaskNode Constructor
The constructor for MTCTaskNode focuses on setting up numerous parameters for the task. It uses a lambda function to declare parameters, which allows for efficient configuration of the task without hardcoding values. This approach makes the code more flexible and easier to maintain, as parameters can be changed without modifying the code itself.
The parameters cover a wide range of aspects of the task, including general settings, controller configurations, robot specifications, scene details, object properties, grasp and place parameters, motion planning parameters, and Cartesian planner parameters. Each parameter is declared with a name, default value, and description. This thorough parameterization allows for tuning of the task’s behavior without code changes, which is particularly useful for robotics applications where many factors might need adjustment based on the specific robot or environment.
setupPlanningScene Method
This method is responsible for setting up the planning scene for the task. It creates a planning scene interface and retrieves various parameters set in the constructor. The method handles the creation of collision objects for the table (if enabled) and the object to be manipulated. It sets their positions, dimensions, and adds them to the planning scene.
This method also includes error handling, logging failures and throwing exceptions if objects can’t be added to the scene. This setup of the planning scene is important as it defines the environment in which the robot will be operating.
doTask Method
The doTask method is responsible for planning and potentially executing the pick and place task. It creates the task using the createTask method and attempts to initialize and plan it.
If planning succeeds, it publishes the solution for visualization.
If the ‘execute’ parameter is set to true, it also executes the planned task. The method includes comprehensive error handling and logging, providing detailed information about the success or failure of the task planning and execution.
createTask Method
The createTask method is the core method where the task is defined. It creates a new Task object and sets up various properties and stages for the pick and place operation. The method is divided into several sections, each corresponding to a different phase of the pick and place task:
- It sets up the task properties and loads the robot model.
- It creates planners for different types of motion, including a pipeline planner for complex movements and a joint interpolation planner for simple motions.
- It adds a stage to capture the current state of the robot.
- It creates stages for opening the gripper, moving to the pick position, and performing the actual pick operation.
- The pick operation itself is broken down into several substages, including approaching the object, generating grasp poses, closing the gripper, attaching the object, and lifting the object.
- After picking, it adds stages for moving to the place position and performing the place operation.
- The place operation is also broken down into substages, including lowering the object, generating place poses, opening the gripper, detaching the object, and retreating.
- Finally, it adds a stage to move the robot back to its home position.
Throughout this method, various parameters set in the constructor are used to configure each stage of the task. This makes the task highly configurable and adaptable to different scenarios.
Main Function
The main function is the entry point of the program. It initializes ROS 2, creates an instance of the MTCTaskNode, sets up a multi-threaded executor, and runs the task. It includes error handling to catch and report any exceptions that occur during the task execution.
After the task is complete, the main function keeps the node running for visualization purposes until the user terminates the program.
That’s it. Keep building!